The vagueness of AI discourse makes implementing AI and measuring its success a daunting goal for business leaders. How can they escape abstract catchphrases and hype to pinpoint functional, ROI-driving applications of AI in supply chain?
A practical approach involves AI diversification – strategic investment in different AI capabilities that perform distinct functions while operating collaboratively. AI diversification involves building a portfolio of capabilities that constantly evolves and grows to mitigate risks, optimize decisions, and maximize value.
How to use AI in supply chain
Many people have some idea of what AI can do for supply chain and retail planning. It can forecast demand, optimize inventory, improve replenishment – the list goes on.
But what is it, and how does it achieve all of this?
Amy Webb of NYU classifies AI as “having a computer do things that normally require human intelligence.” This definition gives us a wide through which to consider how AI algorithms can solve tangible business problems. When it comes to supply chain planning, these algorithms belong to four major categories.
- Heuristics
- Optimization
- Machine learning (ML)
- Gen AI
There are nuances and subbranches in the classifications above. For instance, gen AI is technically a subset of machine learning. However, given the interest it has generated lately and as a nod to its focus on natural language and reasoning, I’m distinguishing gen AI from “specialized AI,” the computationally oriented algorithms listed in the first three bullet points.
I often hear arguments about which of these algorithms is better or if the advent of one makes another obsolete. Such arguments confuse and distract business leaders. Think of each of these algorithms as a tool in a toolkit. They are your wrench, hammer, screwdriver, and pliers. One is not a substitute for another. I could use a heavy wrench as a hammer to pound a nail into a wall, but it’s not the best use of the wrench. Likewise, it’s important to know the context in which you apply each type of AI so you can use the right tool for the right job.
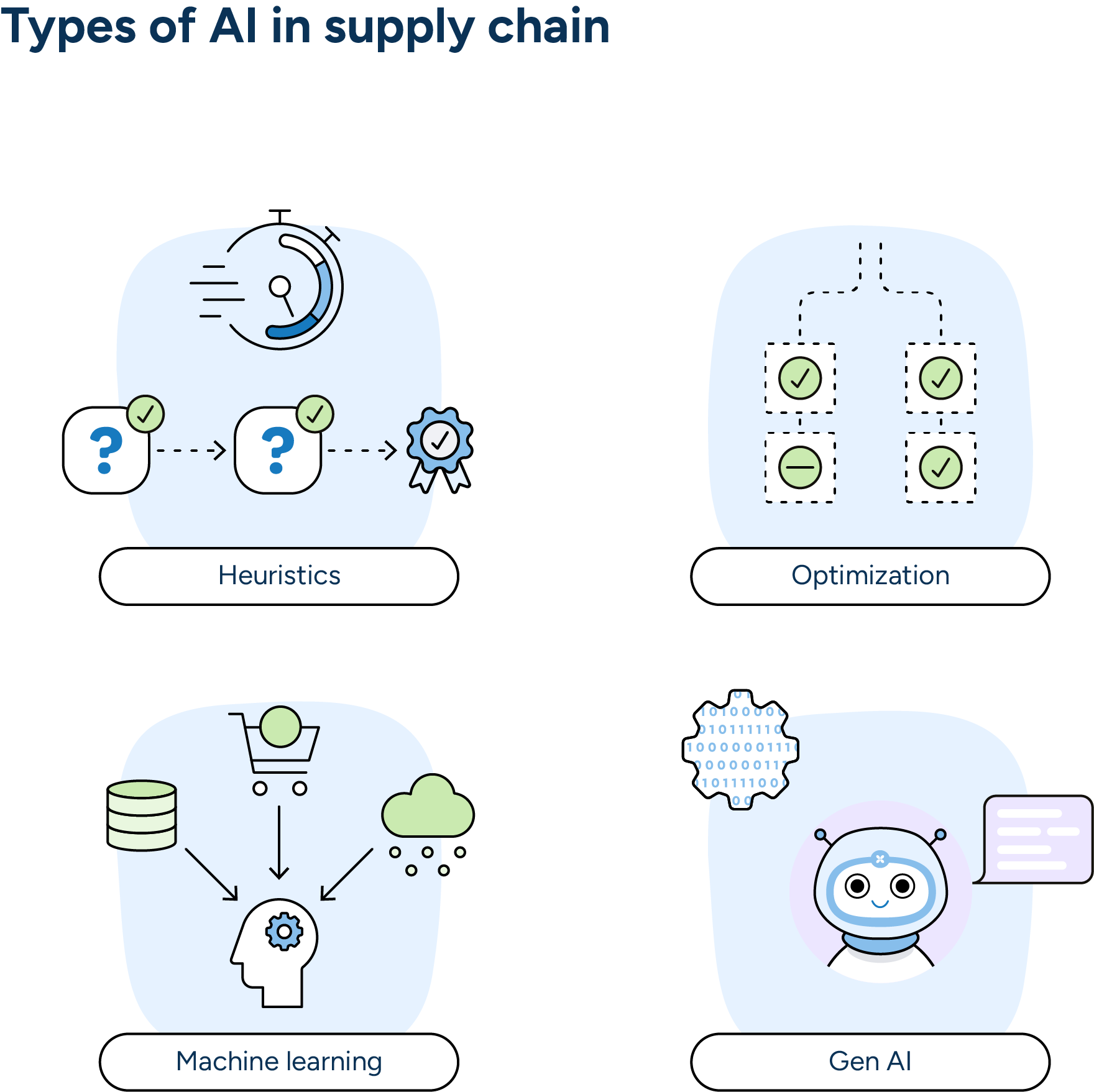
Let’s address each type one by one.
1. Heuristics
Heuristics are rules-based engines that provide directionally correct responses to business problems. They answer a set of questions sequentially, simplifying planning by weeding out options that do not serve particular goals or priorities. By nature, heuristics run fast. Accordingly, they are apt for situations that are less constrained or only require a directionally correct answer.
For instance, one U.S.-based RELEX customer has 30 different distribution centers. Each item travels from the supplier through a predetermined path of DCs, meaning the company does not need to decide where to send stock. This minimizes constraints, so the company can easily employ heuristics to make fast, accurate planning decisions that balance lead times and batch sizes.
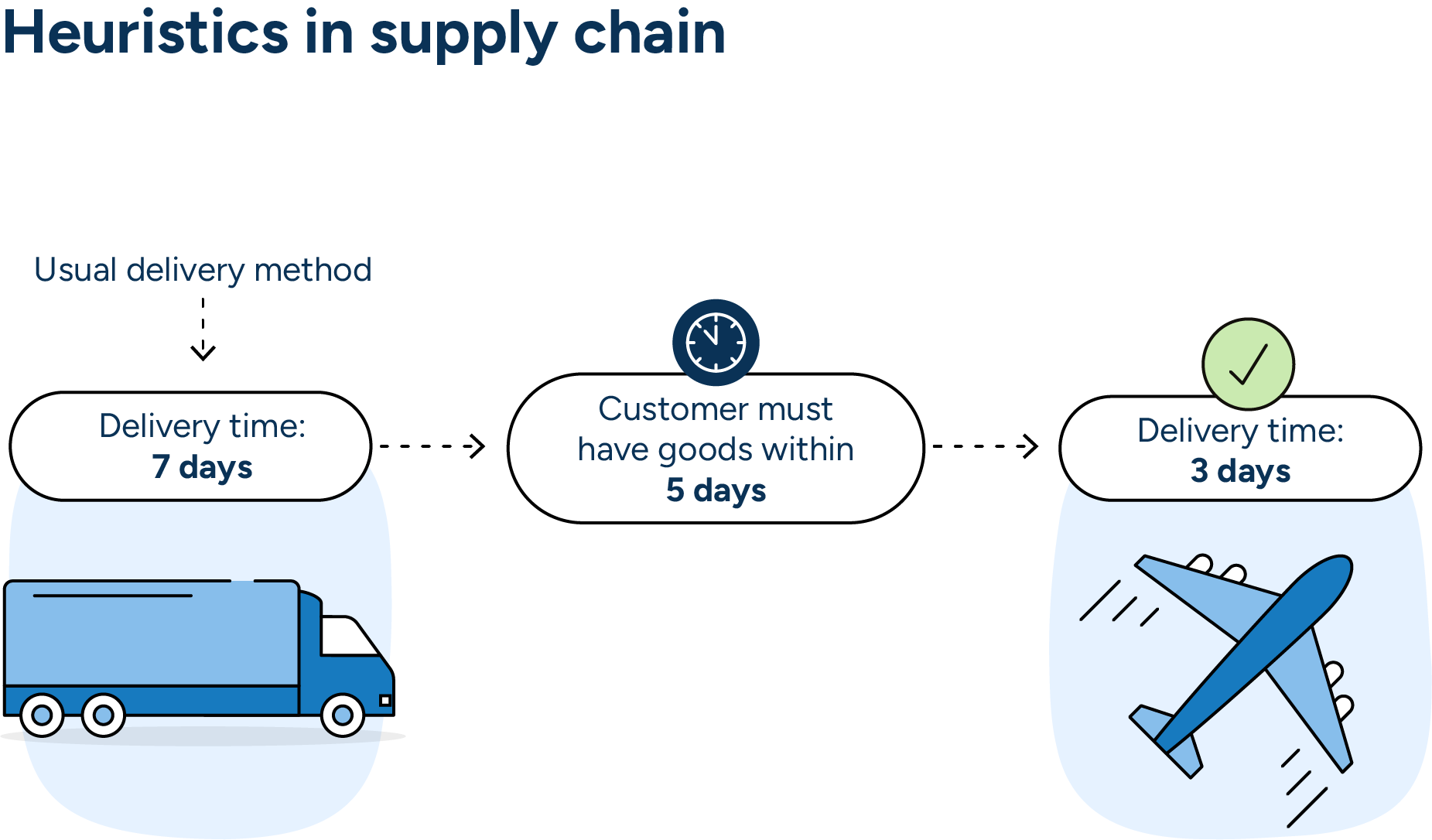
Let’s take a different example. Suppose I’m a distributor that automatically selects trucking as a primary mode of transportation and uses air as a secondary option. I may, however, create a rule that switches to air if the time it takes to get the product to market by truck is longer than what the customer expects.
Heuristics are also great for creating rough cut plans. By considering the most important constraints, they simplify and accelerate the planning process, providing good starting points for plans that optimization capabilities can refine later.
2. Optimization
Mathematical optimization is a branch of applied mathematics that deals with finding the best possible solution from a set of available alternatives, as per deepai.org. Supply chain planning in highly constrained environments, such as manufacturing, requires complex tradeoffs, making it an ideal candidate for optimization.
For example, say you produce mid-grade and high-grade frozen French fries and sell them to quick service restaurants. You have two customers. Customer A will only accept high-grade fries. Customer B opts for the less expensive mid-grade fries but will accept the high-grade option if it does not incur additional costs.
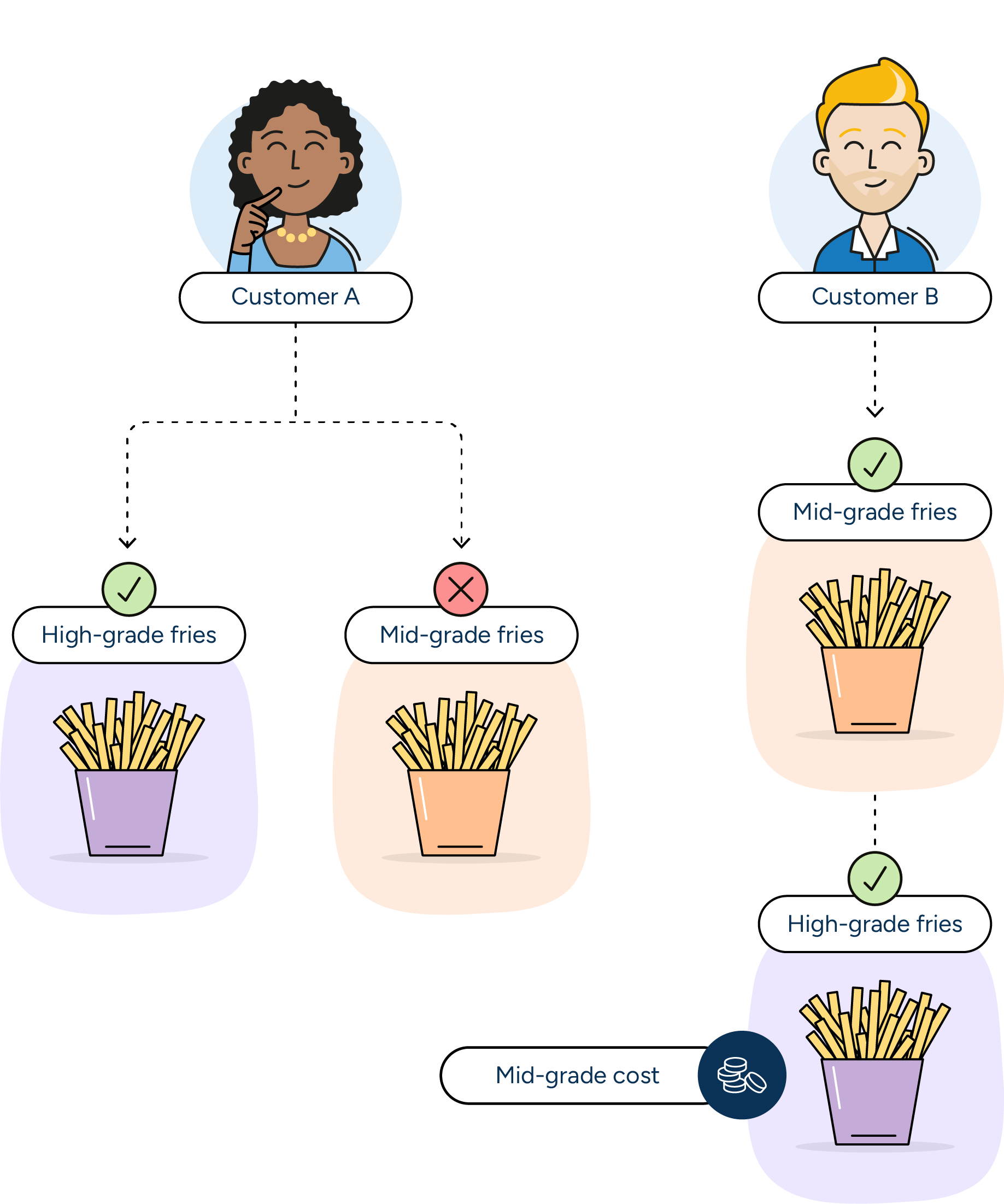
When the customer orders come in, you are out of mid-grade fries, but you can still offer your high-grade fries to Customer B for the same price. This way, you can realize the revenue at the cost of compromising on the margin.
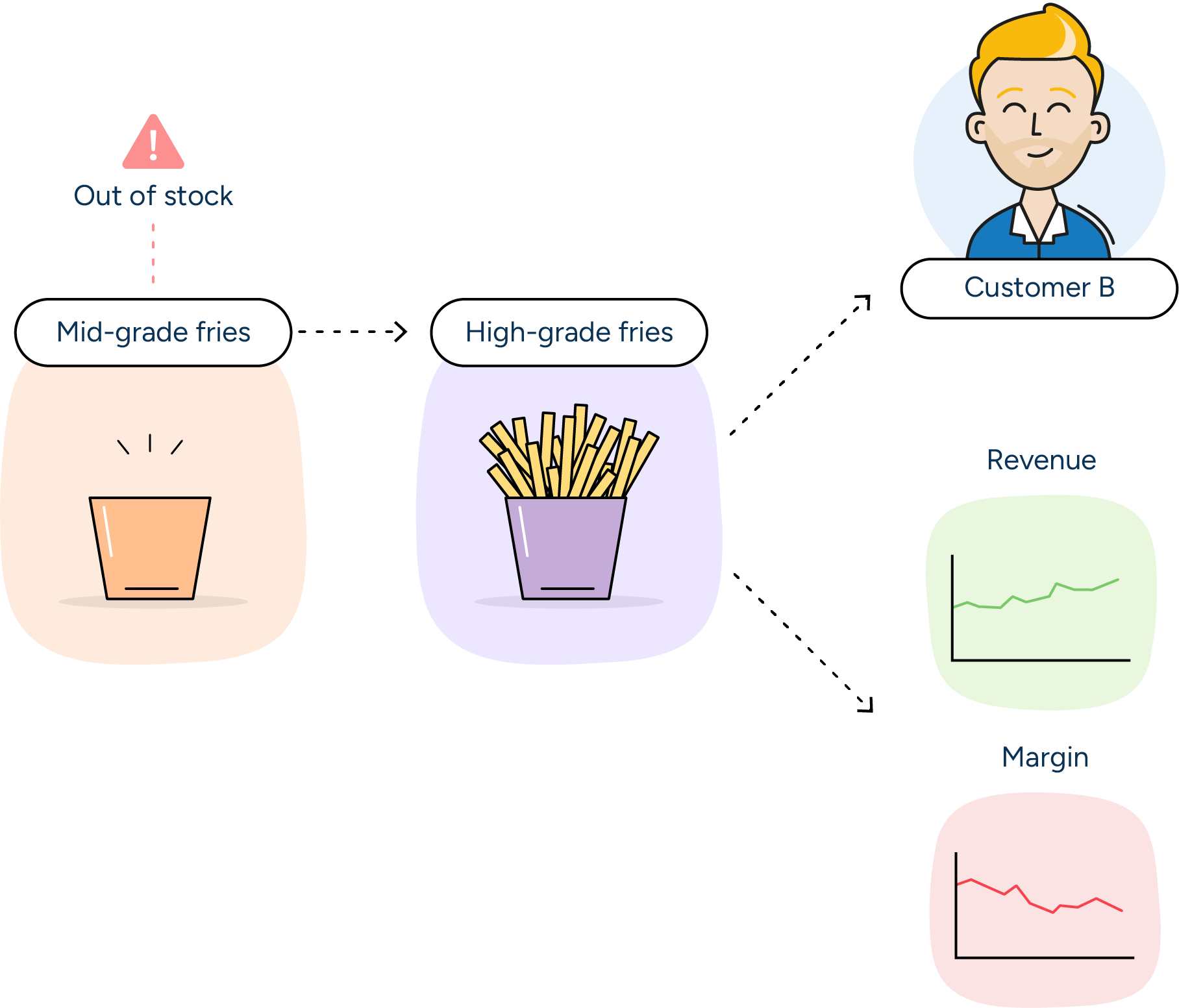
However, you still have Customer A’s high-grade order to fill. Hence, the benefits of trading up Customer B for higher-grade fries must be balanced against the anticipated demand from Customer A.
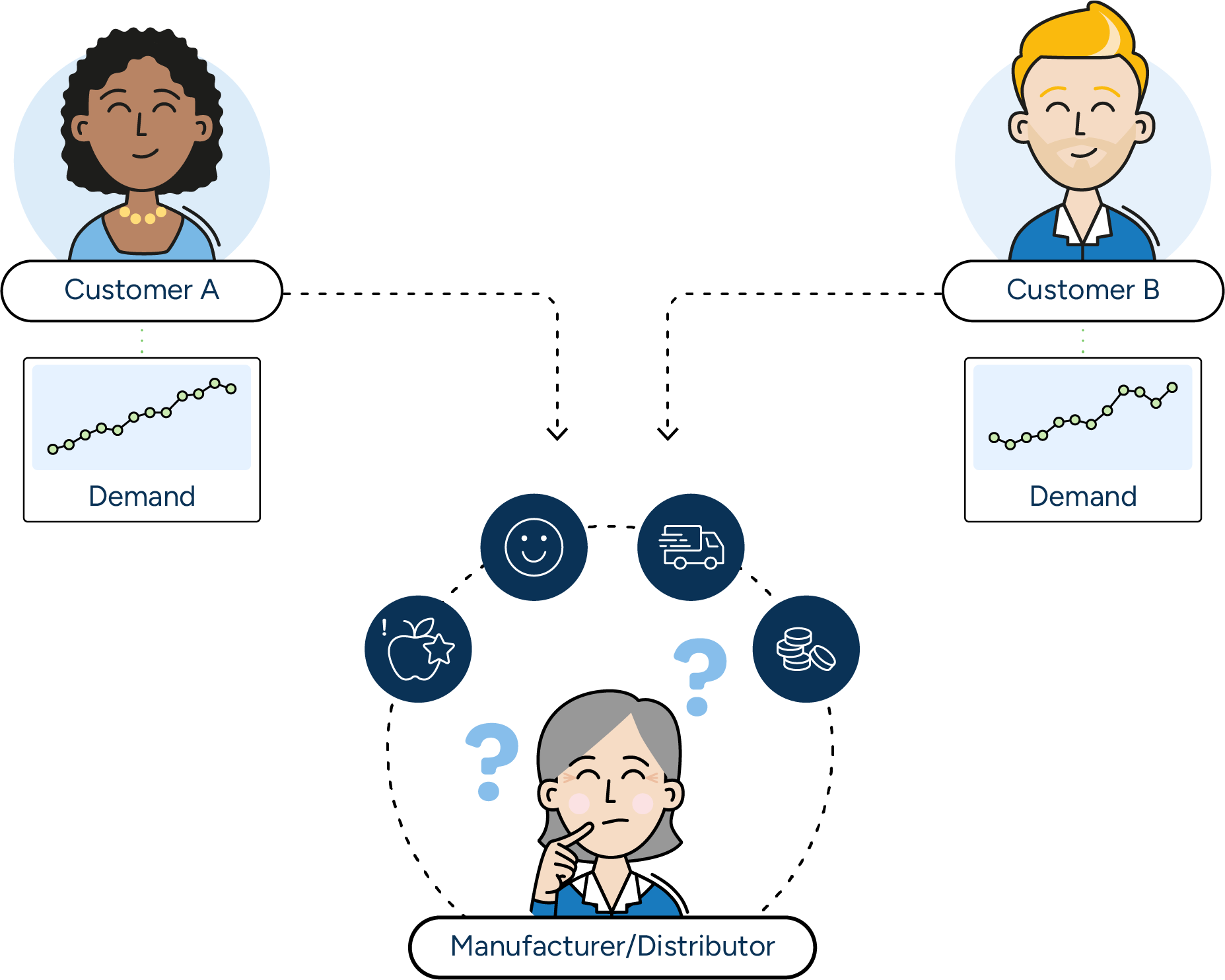
This is an extremely simple example of the kind of tradeoffs optimization excels at balancing.
However, real business involves far more complex decisions concerning a variety of constraints – raw materials, capacity, customer priority, etc. These types of use cases lend themselves very well to optimization, which analyzes these constraints, considers business objectives (like maximizing revenue or minimizing costs), and processes available data rapidly for optimal trade-off recommendations.
Tip: There are some similarities between the types of problems to which heuristics and optimization can be applied. At times, I hear debates about which approach is superior, but it should not be an “either/or” debate. It should be an “both/and” answer, picking the best tool for the circumstances or using a combination of the two.
3. Machine learning (ML)
Machine learning is “the use and development of computer systems that are able to learn and adapt without following explicit instructions, by using algorithms and statistical models to analyze and draw inferences from patterns in data,” as per Oxford Languages. This method is best used to draw correlations between various data streams to make predictions.
Practical examples for this include sensing shifts in demand or performing root cause analysis.
For example, when it comes to demand planning, machine learning algorithms help planners across industries tackle the sheer amount of information global supply chains generate. Unlike clunky spreadsheets, machine learning-based forecasting solutions analyze and distill large data pools into manageable insights for more accurate demand forecasts and data-driven decisions. What’s more, machine learning algorithms learn from and refine outcomes over time; the longer they are used, the better their output.
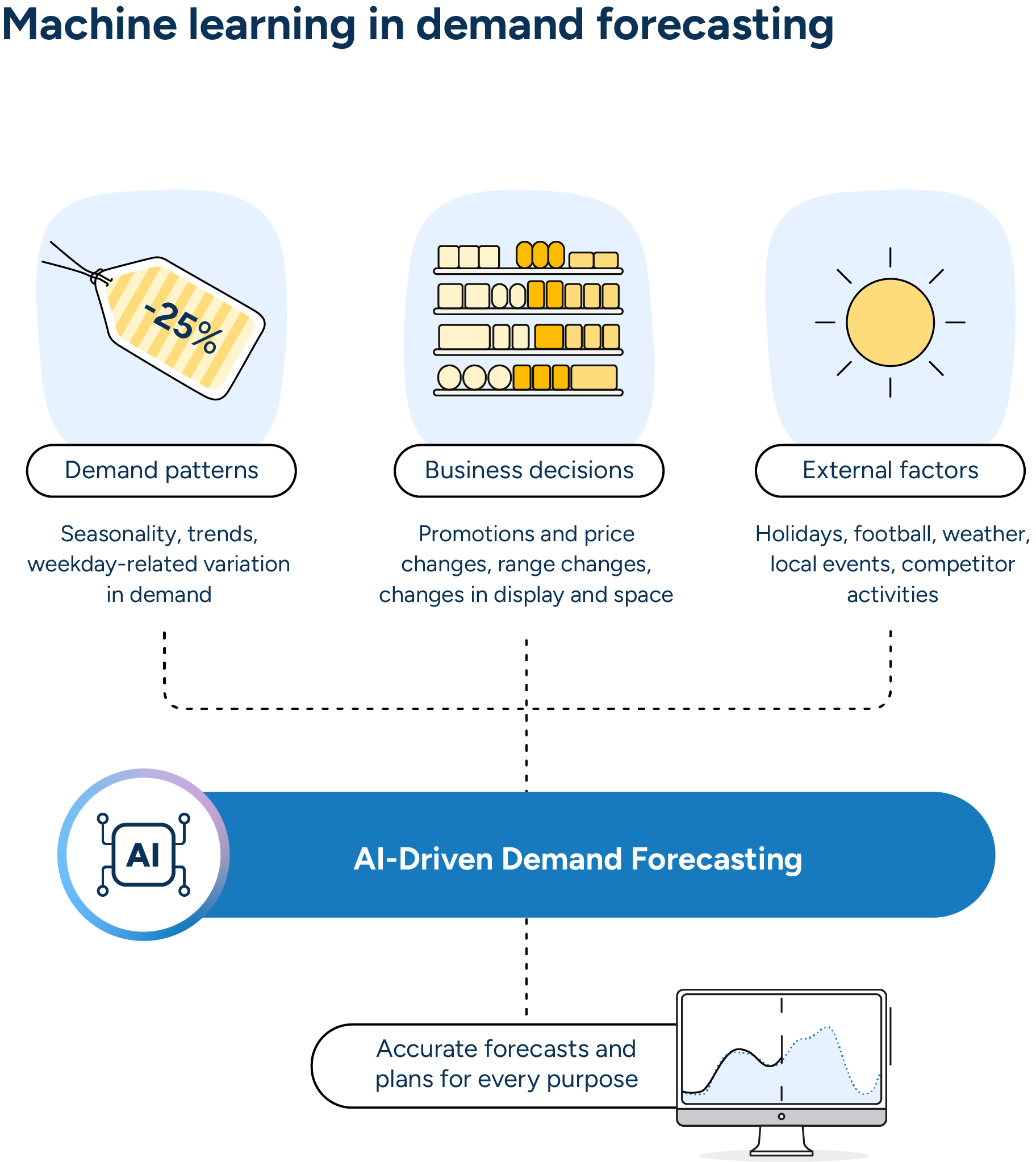
Demand sensing takes these analytical capabilities to the next level, using machine learning to integrate current data from across the supply chain (including retail POS systems). This makes it perfect for retailers and fast-moving consumer goods (FMCG) companies who need to keep up with rapidly shifting market trends or sudden weather events. For instance, if there’s going to be a major sporting event, there may be an increase in demand for soft drinks and alcoholic beverages. If it’s going to rain all next week, sunscreen demand may drop while umbrella sales spike. Using machine learning, companies can predict whether they will sell more or less of a product. ML-based predictions inform the optimization capabilities that determine how much of the product to replenish in each location.
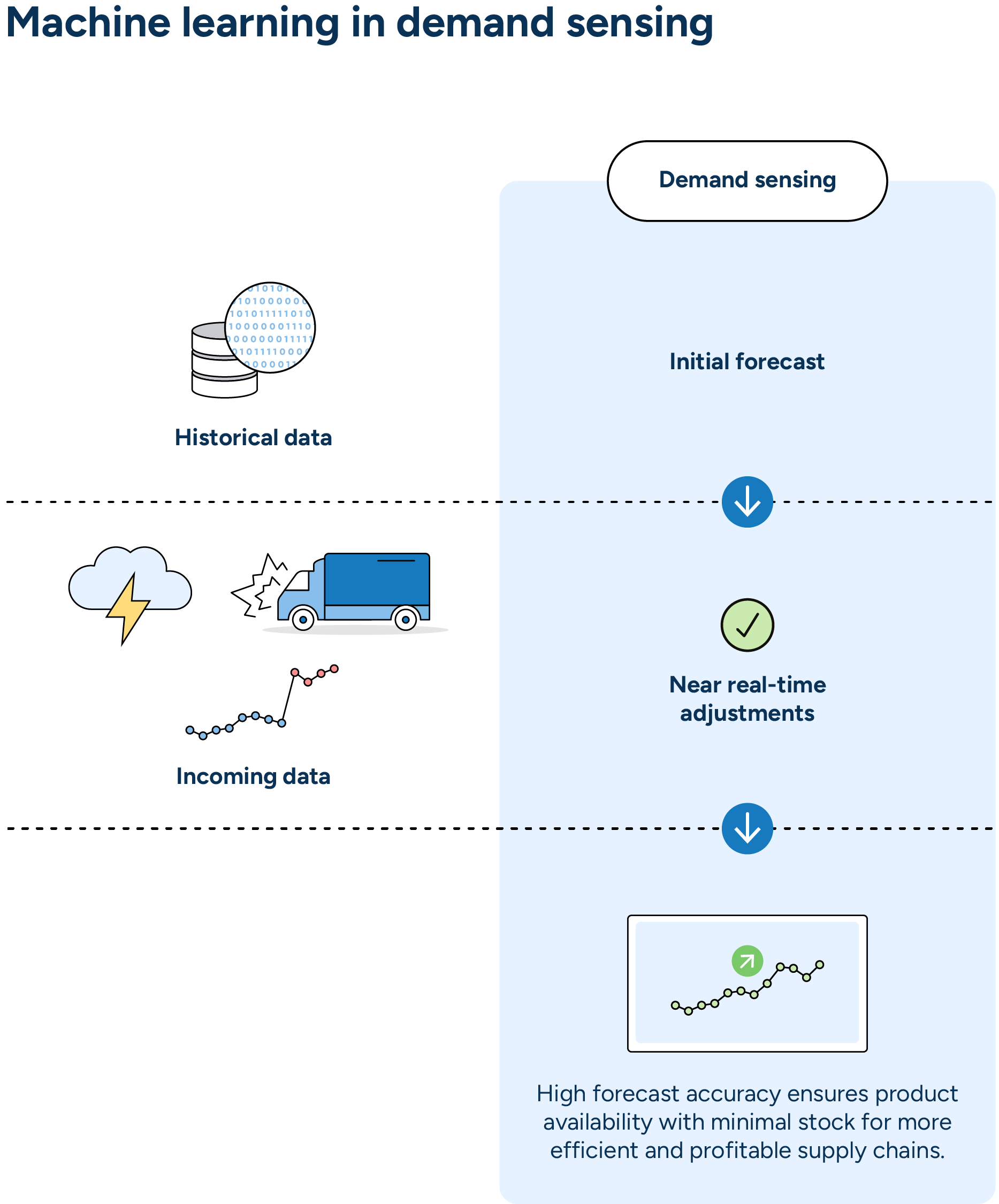
Machine learning also aids in root cause analysis of the gaps between planning and execution. Often, planning recommendations – such as how much to make, procure, and deliver – don’t get implemented correctly in the real world. This may stem from discrepancies between planning assumptions and the actual status of contributing factors like lead times, costs, or transport availability. Planning parameters must be constantly adjusted by being tested against real-world data. The ability to tap into various internal and external data streams and correlate them comes naturally to ML algorithms, making them uniquely suited to situations that call for this kind of analysis.
Geek alert! I often get asked whether optimization as a technique will become obsolete with the growing prominence of ML. On the contrary, optimization will survive and thrive; there is no machine learning without optimization. This is because an ML model is refined by calculating a “loss function” and running optimization to minimize that loss. (A loss function quantifies the difference between the model’s predictions and the actual output, providing feedback on the model’s accuracy so it can adjust and improve going forward.) Best-in-class planning solutions use machine learning to predict and optimization to decide.
4. Gen AI
The new kid on the block is gen AI. Coursera defines gen AI as an algorithmic approach, trained on existing data, that “allows users to input a variety of prompts to generate new content.” While the principles have been around for a while, advances in high-end chips and the rise of cloud computing make the technique accessible for practical applications.
With all the excitement, it is crucial to remain realistic about what gen AI can and cannot do. For instance, large language models (LLMs) are the most common use case of gen AI. They are poor at performing calculations, but they are very good at looking at a string of words and predicting what the next word will be (as many have experienced through the likes of ChatGPT and Gemini).
Supply chain systems are inherently complex. Heuristics, optimization, and machine learning aid with computations, but context and collaboration are equally important in supply chain decision-making. This is where gen AI comes in.
We are starting to see the use of LLM-powered chatbots as AI assistants, ushering in significant advances in productivity and user empowerment. A supply chain assistant should pull from an established knowledge base of industry- and solution-specific best practices and draw insights from the data in context. Making this information easy to access and understand accelerates employee onboarding, smooths daily operations, and provides more opportunities for continuous learning and process improvement.
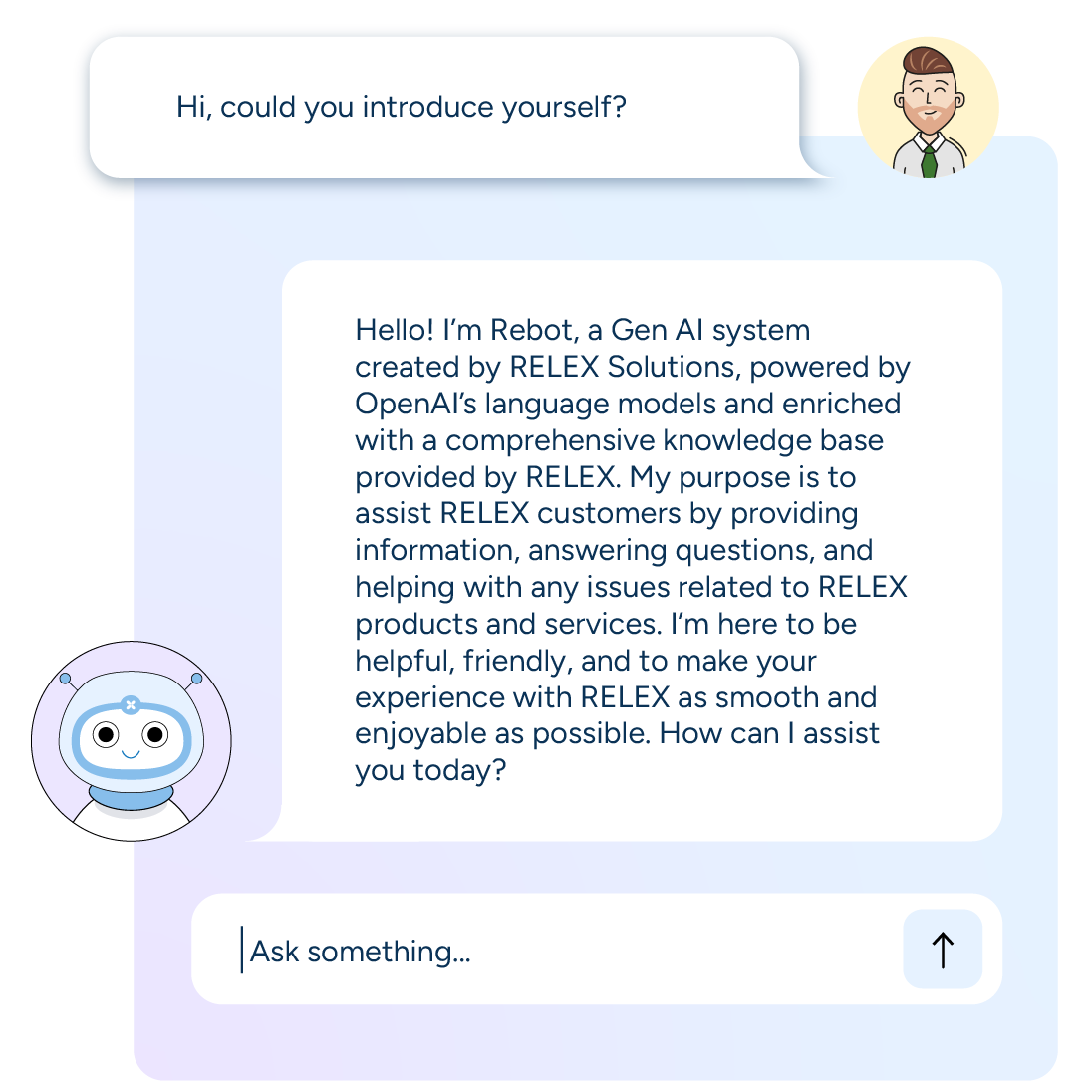
Looking ahead, these systems will evolve into fully fledged AI copilots that provide company-specific recommendations and proactively assist planners in anticipating and countering market disruptions and shifts.
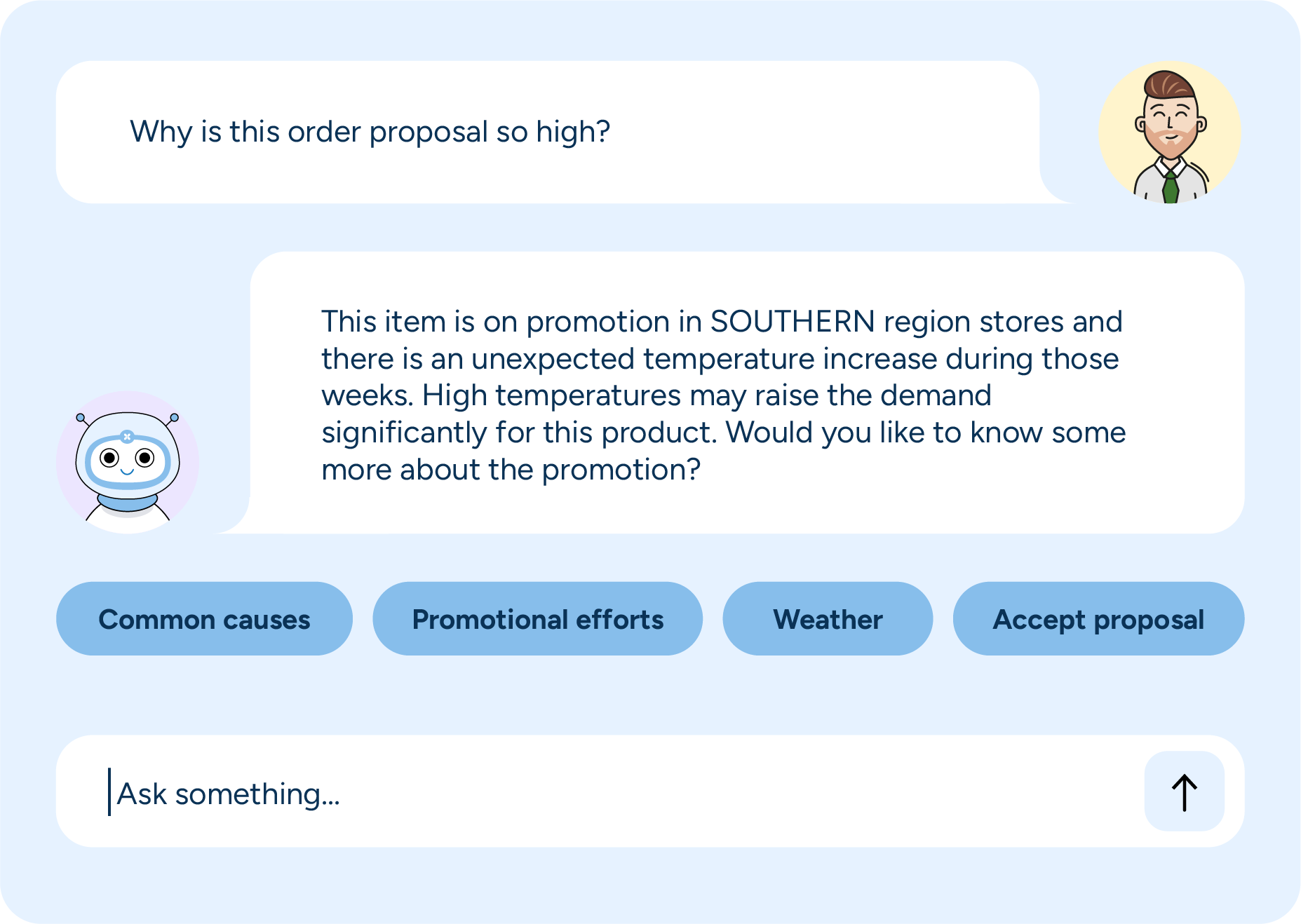
How AI diversification benefits supply chains
Thoughtful AI implementations do more than just check a box. They create efficiency and productivity gains for users, who can improve and speed up decision-making. Those decisions set inventory targets in the correct proportion to demand, fulfilling customer needs while keeping inventory levels under control. By avoiding excess stock, the strategic use of AI limits the prevalence and impact of issues like obsolescence and spoilage, which supports sustainable supply chain practices and frees up working capital for better margins.
Business leaders should view AI as a collection of algorithms and apply techniques to specific problems in a practical fashion rather than doing AI just for the sake of doing AI. Fortunately, there are plenty of tried-and-true applications with the ROI to prove their efficacy.