Benefit from forecast data in your planograms
Jan 11, 2024 • 5 min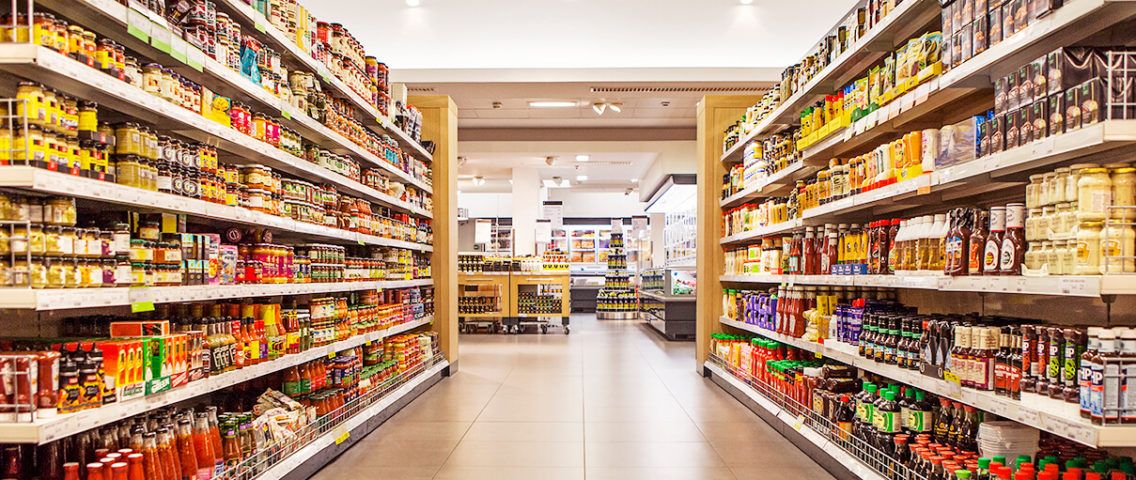
One question that seems to come up constantly when discussing space planning is, “Can we use forecast data when making the planograms?”
The most common answer given by most planogramming systems seems to be, “If you give us the forecast data at the weekly product-location level, then yes.” This means that one should turn the forecast data into weekly averages and then build the planograms based on that.
The problem with this answer is that it doesn’t make sense since it doesn’t address the real questions:
- How do you find a way to combine forecast and historical data?
- How do you manipulate forecasts into averages?
- Can we be sure the forecasts are accurate enough?
In this blog, we outline the challenges and propose how these can be overcome.
The logic behind using forecasts in planograms
A planogram must represent every trading day in the week, so usually, the first port of call would be to turn to one’s sales history and average it out. However, retail has moved away from generic planograms towards cluster- and store-specific planograms because we’ve learned that no two stores are the same. Likewise, we should address the fact that no two days are the same – something everyone who has worked on the front line in retail knows too well. Rather than using past sales in planograms, you can produce forecast-driven planograms that accurately reflect trends and seasonality in demand by using forecast data.
So, as well as having enough bake-at-home pizzas on the shelf for an average day in each store, that store next to the big auto factory can be ready for an increased demand on payday Friday (if you’re a fan of pizzas)!
The challenge with historical data
When we use historical data to build planograms, we are making a crude projection that the next 12 weeks will, on average, be like the last 12 weeks. Of course, we all know that’s not the case. By considering demand forecasts, we can upgrade these projections and make them more powerful. For example, historical data might not include weather fluctuations, trends, seasonality, etc. Here, forecasts can prove to add significant value.
So, suppose we have intelligent forecasts 12, 52 or 104 weeks into the future that give due weight to historical data. In that case, these are at least as valuable as our historical data and likely even more.
Most conversations I’ve had about using forecast data in planograms were focused on using it like a new historical metric, the same way we would talk about incorporating consumer loyalty data. The more I work with forecasts, the more I realize it would be foolish to take forecasts built to project the future and merge or dilute them with historical data. After all, the forecasts have already been drawn on historical data; we are taking backward steps by performing a merge or average.
Why you shouldn’t rely on weekly averages
Planograms have historically used weekly sales averages (undoubtedly because the market-leading space planning solutions are entering their 4th decade of life). That weekly average is then divided by the number of opening days to get the average daily sales. The next step has naturally been to use this average daily figure to determine how many days of coverage are on the shelf.
I reflected earlier that nobody in retail can remember ‘an average day’. Why are we then averaging averages and building the primary interaction point with the customer around these approximations?
Consider this typical example: In light blue, we see the daily demand for a product in a single store, and dark blue shows the average sales. Note how different almost every day is from the average.

With two deliveries per week, placing 3 ‘days of supply’ on the planogram would be standard practice. On average, daily sales are 14 units, so our 3 ‘days of supply’ are 42 units. Looking at the example above, if we re-shelve on Friday morning after delivery the same morning, we would have an empty shelf midway through Saturday.
Now, it’s reasonable to consider that we’ve used average weekly data in the past to keep data volumes down and smooth out all sorts of peaks and troughs that we wouldn’t want to base our planning on. I’m a big fan of rough calculations to see if something makes sense, but it’s time to start thinking about approaching space planning more scientifically.
Can we be sure the forecasts are accurate enough?
The most astute retailers will use a period reflecting the category review frequency when using historical data in planograms. So, they will use the previous 52 weeks of performance data for a category that is changed once a year, the previous 12 weeks of data for one that lasts 12 weeks, and so on.
You must do the same thing when using forecast data in planogram production. So, for a seasonal planogram to last 12 weeks, you need 12 weeks of forecast data.
Many retailers would naturally ask, “Is the forecast accurate enough to rely on it 12 or 52 weeks into the future?” The only proper way to answer this question is to test it empirically. Still, we can be confident that if we compared 12 weeks of forecasted sales (for weeks 13-25) to actual sales (for weeks 13-25) and then to averaged historical sales (for weeks 1-12), the forecasted sales would be no further away from the actual than the average – and I’d be very confident the forecast would be a lot closer. More about this can be found in our forecast accuracy guide.
Unify your planning and benefit when building planograms
In a world with exceptionally powerful technology and systems that create forecasts and smooth out anomalies, it’s suddenly a lot easier to bring something different and value-adding into your planograms and be thinking ahead. Unifying planning across multiple functions is not only applicable to planogramming – it benefits store and macro-space planning, promotions, workforce, ordering, and replenishment. Our vision is to unify retail planning and challenge the industry’s siloing of things like space and supply chain planning by breaking down the redundant boundaries between them. Retail is changing. You run the severe risk that while you miss a trick, your competitors won’t.