Increasing labor efficiency in stores and distribution centers with inventory management solutions
Sep 6, 2024 • 9 min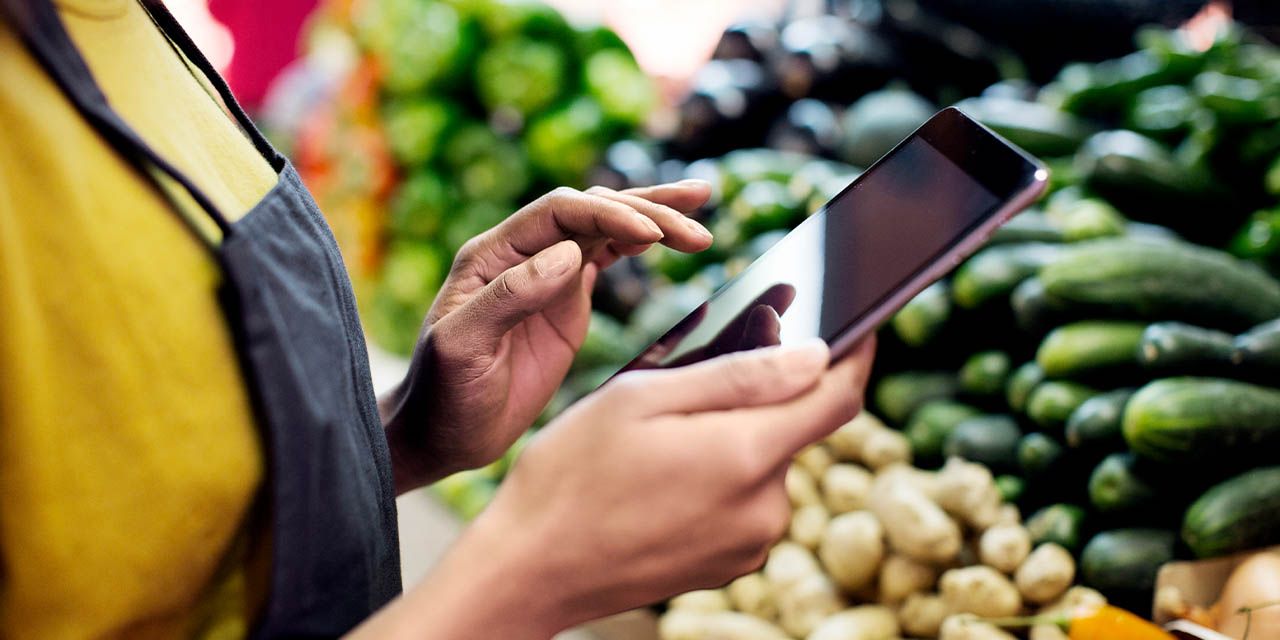
Labor efficiency depends on inventory transparency
Labor efficiency is a cornerstone of retail and supply chain success. However, many retailers and distribution centers face significant challenges, including high labor turnover, manual planning processes, and inefficient resource utilization.
In today’s market, labor has added more strain to retailers’ operations. Whether it is the rising cost of labor, retention of the more experienced workforce, or needing to onboard a different profile of workers onto complex systems or processes, all these scenarios and more are increasing in frequency, hurting the efficiency of the supply chain.
However, at the same time, many retailers that invest in more advanced planning solutions are focused on benefits to inventory, spoilage, and sales but neglect to take advantage of the potential improvements these solutions can provide to the efficiency of their workforce. Combining replenishment and labor planning enables optimized deliveries during low-demand hours and days, reduced wasted effort, and improved customer service despite workforce shortages.
Given that optimizing labor efficiency is essential to maintaining profitability and ensuring customer satisfaction, we believe further margin improvements can be made by aligning AI solutions to workforce optimization initiatives. RELEX provides retailers with a wide variety of data sources to forecast and plan labor and enables them to find new efficiencies where they did not exist before.
Here, we have outlined seven common examples of where AI solutions drive significant improvements to product availability and inventory levels in-store but can also be leveraged further to deliver labor efficiencies when aligned with labor planning, training, and colleague engagement. We explore practical ways to leverage your existing or planned solution implementation to deliver additional benefits.
Central automation leads people to focus on minimizing supplier out-of-stock
Retailers are all moving to more AI-based solutions for central forecasting and replenishment because AI brings massive performance improvements to crunching large datasets and accurately and automatically proposing intelligent orders.
Depending on the manual work level before moving to AI-based solutions, this shift should release the central forecasting and replenishment teams from the more menial actions. If the teams are not given clear role description changes to more value-added responsibilities where humans excel, they tend to default to overly zealous reviews of the AI outputs, making inconsequential tweaks to orders.
We always advocate for clear role updates whenever new AI solutions are introduced and for evaluation of the areas in the teams that add real value to the business. One area often under-invested in is relationship management and collaboration with suppliers.
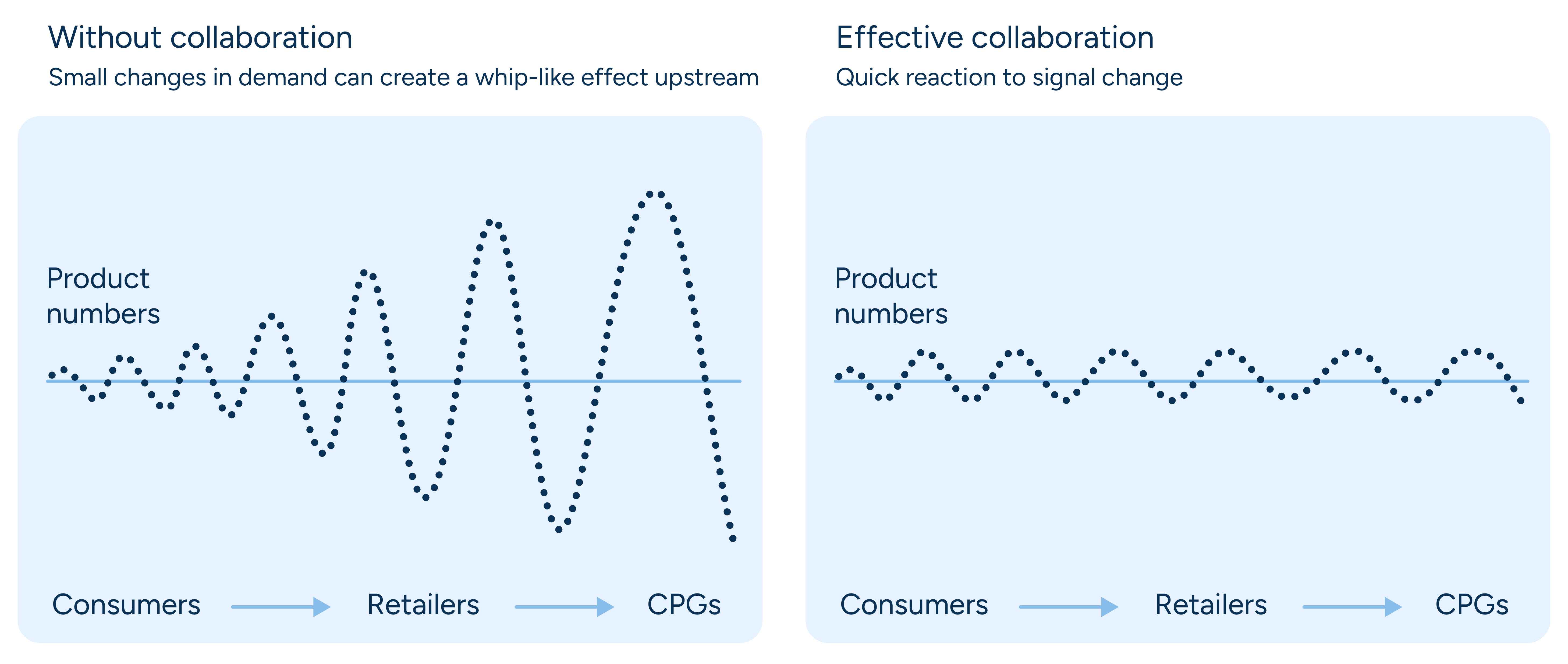
Our Supply Chain Diagnostics analysis has shown that supplier delivery shortages are the leading cause of out-of-stock for many retailers. More time invested in joint planning around new product launches, promotions, price changes, and other supply disruptions means central teams can proactively align on order volumes to improve supplier performance or find ways to react to supply disruption via alternative sourcing in a much more proactive way.
The collaboration with RELEX in terms of supply chain transparency and diagnostics has been transformative for us. Everyone working in the supply chain should familiarize themselves with this.”
– Tuomas Tiilikainen, Chief Supply Chain Officer at Stockmann.
Smoothing of ordering through the distribution center
Customer demand often manifests in peaks and troughs with a regular weekly, monthly, and seasonal profile. Retailers will attempt to align replenishment processes to these profiles, but this results in distinctive discrepancies in day-to-day staffing requirements and labor costs. This can lead to issues at both extremes:
- During peak days, staffing is pushed to its capacity, resulting in staff working longer labor hours and perhaps being overworked. In some circumstances, retailers may need to resource these days with a temporary or exceptional workforce during more expensive times. In the worst case, if a pick is not made, the store supply is compromised, which can lead to lost sales and unhappy customers.
- During quieter days, residual capacity for picking/receipting goes wasted. Retailers maintain a minimal staff level that may have some wasted utilization and inefficiency.
The most effective way to deal with these demand peaks and troughs is to smooth your replenishment strategy rather than chasing the demand. Plan your stockholding so that goods with storage space, shelf-life, and appropriate upcoming demand are pulled forward from peak days into sparser periods. Examples include:
- Pull your weekly replenishment from a traditional heavy weekend toward Monday/Tuesday.
- Early pre-seasonal filling for Christmas peak weeks in December.
Limit-based delivery flow smoothing minimizes stockholding targets on over-capacity days and offsets this directly with increased targets in earlier periods.
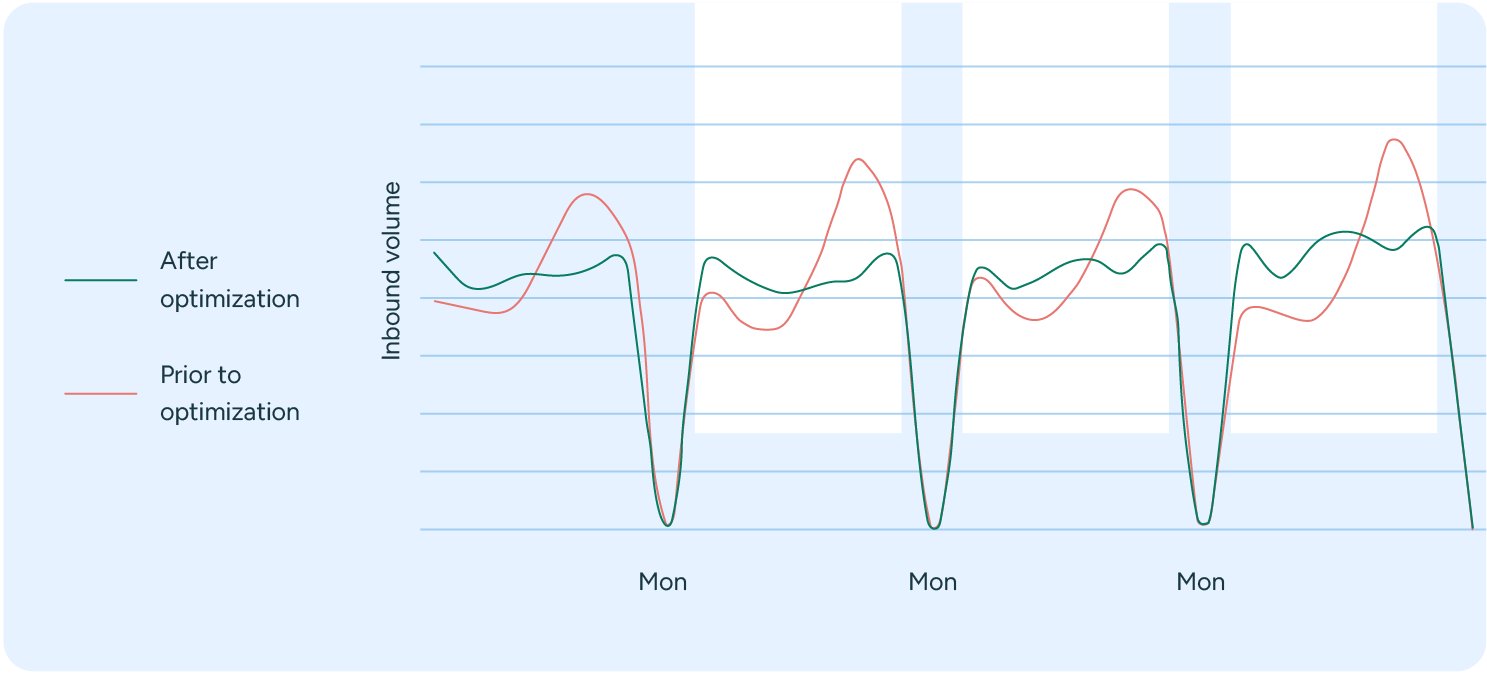
Smoothing downstream store replenishment can target DC outbound limits, smoothing DC pick forecasts. The same smoothing can also be applied on the DC level to attempt inbound smoothing. The net effect is a less volatile, more manageable workload in DCs, ultimately decreasing labor costs and improving labor efficiency.
“Our store personnel now find the deliveries better planned and more manageable, which makes their daily work a lot easier. RELEX’s delivery flow smoothing has quickly proven its value without compromising our other objectives.”
– Gina Hedqvist, Supply Chain Manager at Granngården.
Prioritization of picks in constrained supply scenarios makes DC more efficient
In situations where supply is lower or later than expected, DC staff must often decide how to fair share the scarce stock that is available to pick. This is sometimes a complicated decision, and the optimal decision requires a depth of information on each store’s demand, stockholding, etc.
Automated scarcity suggestions remove this burden and ensure DC picking can react quickly when on-time-in-full (OTIF) failures or unforeseen demand spikes occur. In an optimal set-up, not only does a central allocation for day-0 scarcity situations take place, but proactive adjustments to projected orders should be made for known future shortfalls as well. This has a dual benefit of:
- Providing a realistic DC outbound estimate to DC staff so they can prepare their labor accordingly.
- improving the accuracy of DC inbound ordering to ensure future scarcities are less likely
Order cycle optimization, minimizing pick rows to deliver in the most cost-effective way
Replenishment contracts with suppliers often allow orders to be placed on any day of the week. Although this flexibility is welcome, placing daily orders into the DC is not necessarily optimal. Instead, consolidating demand into larger, less frequent orders can save on administrative, transportation, and handling costs.
It is essential not to restrict ordering frequency without some intelligent control and the ability to react to day-to-day uncertainty. Rigidly cutting down on delivery opportunities can result in poor availability if demand spikes occur. On the other hand, if sales underperform, the DC can end up with too much stock too early, causing unnecessary fluctuations in order handling.
Dynamic order cycles allow you to combine the economies of scale of larger orders with the flexibility of ordering any day of the week. If your order cycle is dynamic, it will first check if you have an order need before placing an order large enough to ensure it is economical. The order cycle (how often you order and on which days) is then changed to account for this exceptional, economical order that has just been placed.
Notably, a dynamic order cycle results in fewer unique order rows, reducing the time spent receiving stock in the DC (or picking stock if applied to stores downstream) and significantly cutting labor costs.
Shelving optimization, using floorplans to plan the delivery of items to make shelving more seamless
Most retailers recognize the value achieved by optimizing store layouts and are investing in new floor planning software or undertaking large refit programs to relay shop floors. The benefits typically associated with these programs involve increased sales or enhanced shopper experience. However, we believe they can also improve shelving efficiency by connecting store deliveries to the floor plan. 40% of labor costs are typically spent handling goods, and improvements made to the end-to-end shelving process can support labor efficiency.
Consolidating product deliveries in the same shelving area can lead to more efficient DC picking due to a focused collection of similar products. It can also reduce the need for store personnel to move pallets and roll cages around the whole store during shelving.
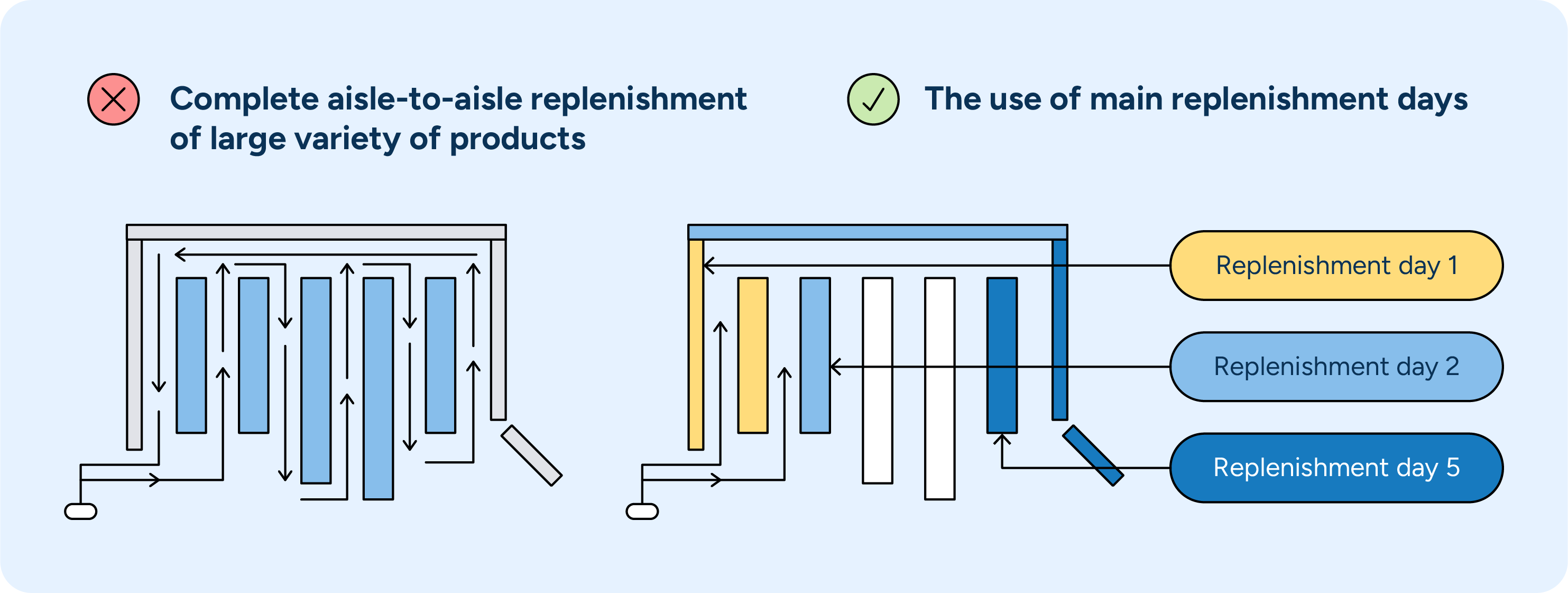
Planograms are optimized typically for sales uplifts but can minimize shelf touches if synced with demand
Planograms provide the point where the end consumer sees and feels all the retailer’s planning and decision-making. As such, space planners labor over planogram creation, balancing consumer experience, brand consistency, and merchandising compliance to ensure they get the space allocation right.
To maximize benefits, most retailers try to move towards store-specific planograms to cater to the individual needs of each store. However, in most cases, they struggle to consistently generate benefits and a business case to justify the investment. With the increase in AI capabilities, optimizing planograms by considering shelving efficiency as an additional variable and other standard merchandising factors is now possible.
By utilizing projected inventory levels from the supply chain as performance data within planogram optimizations, retailers can drive ‘one-touch’ replenishment within stores. This should reduce the amount of delivered stock that does not fit on the shelf the first time, helping improve customer availability and store personnel’s shelving efficiency.
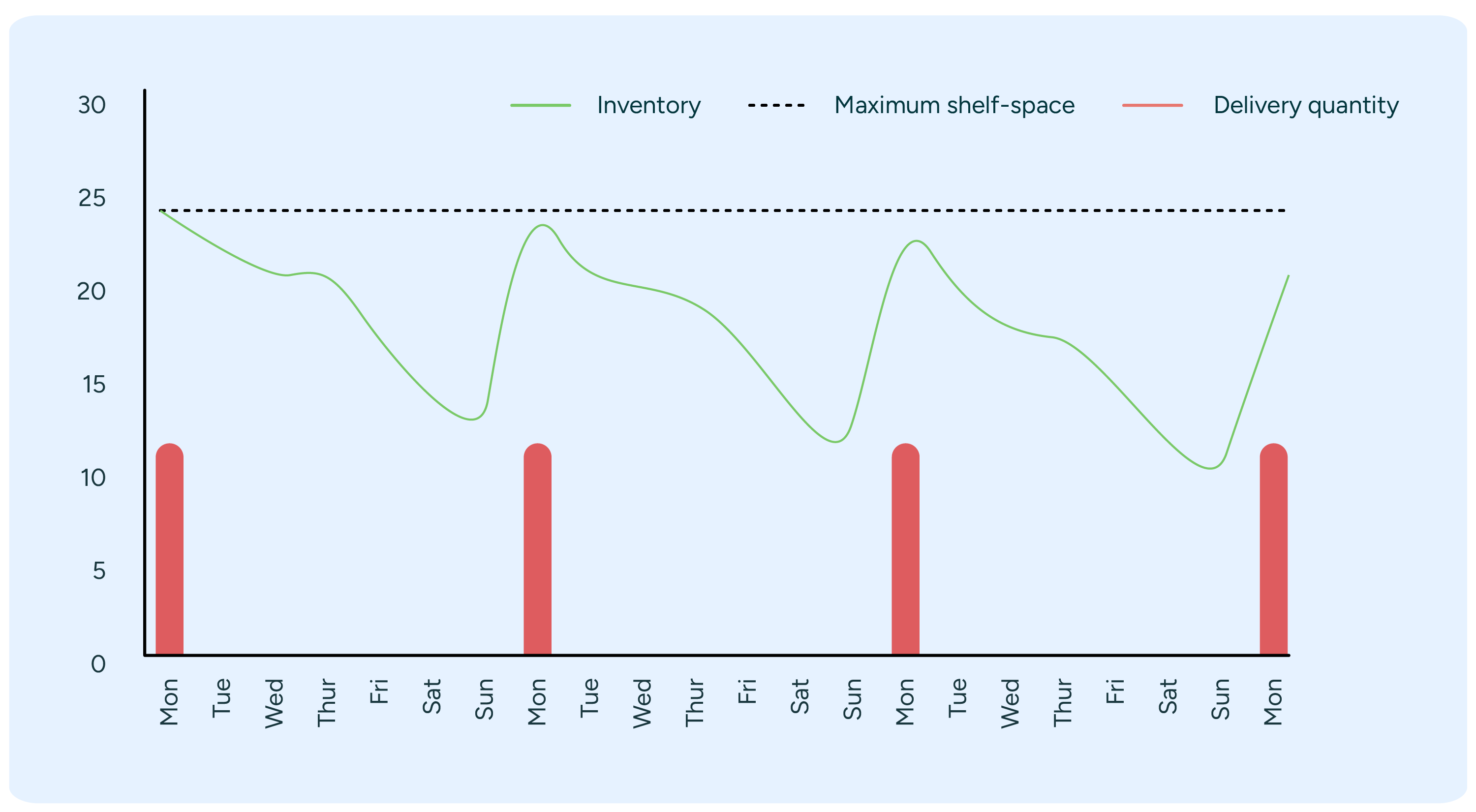
AI assists in store routines to maintain accurate inventory balances
Accurate inventory data is fundamental to ensuring the order accuracy of AI models, but in-store routines to check inventory levels have generally stayed quite basic and standard across entire sections of the store.
We are seeing increasing challenges with inventory drift, partly driven by increased shrinkage due to a multitude of socio-economic pressures, such as the cost of living. As inventory drift challenges persist, the necessity of completing these inventory check routines efficiently increases.
Help is on hand in the form of predictive inventory AI models that forecast unplanned inventory transactions in all their forms. These models can replace or partly replace more classical perpetual inventory records, but they also improve the efficiency of the colleagues in the store doing the count routines.
Predictive inventory models can highlight problem areas and suggest more intensive count routines while suggesting lower frequency checks in more stable product groups. This more targeted routine setting means more time is spent on finding and correcting issues and less on ‘ticking the box’.
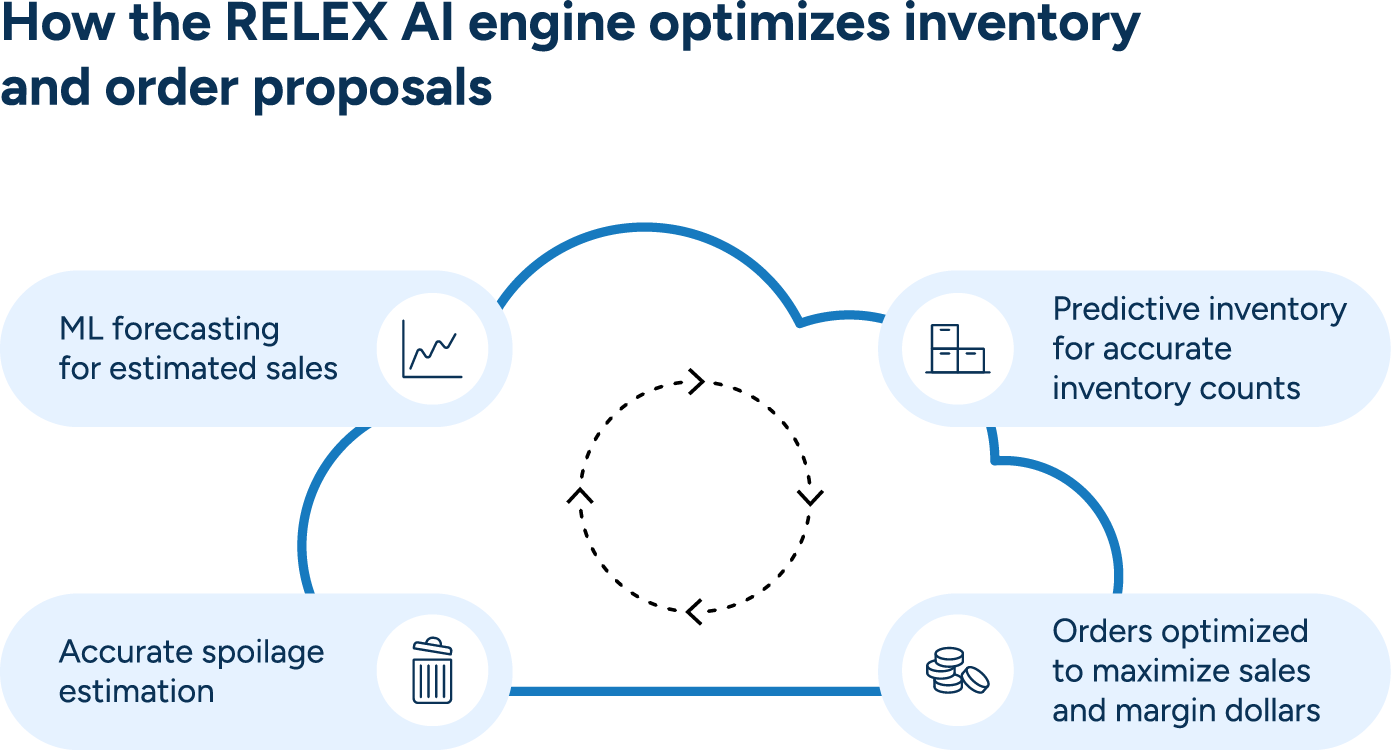
These models, paired with platforms with intuitive and easy-to-use UI, mean that there is less pressure on the store staff to learn to navigate complex systems, so onboarding and training time are radically reduced. It also means that when time is tight and skilled resources are tied up, less experienced staff can execute these critical routines in order of priority.
Increase labor productivity with best-in-class inventory management solutions
In conclusion, leveraging AI-driven inventory management solutions presents a significant opportunity for retailers to enhance labor efficiency in both stores and distribution centers. By automating and optimizing key processes such as forecasting, replenishment, and inventory checks, retailers can improve labor productivity and reduce associated costs while better allocating human resources to value-added tasks.
These improvements not only enhance operational efficiency but also contribute to higher customer satisfaction and overall profitability. All the information can be fed from RELEX to your labor planning system with RELEX Workload Forecasting. As the retail landscape evolves, embracing advanced technological solutions will be essential for maintaining a competitive edge and achieving sustainable growth.