Making your spares management more efficient
May 27, 2008 • 9 min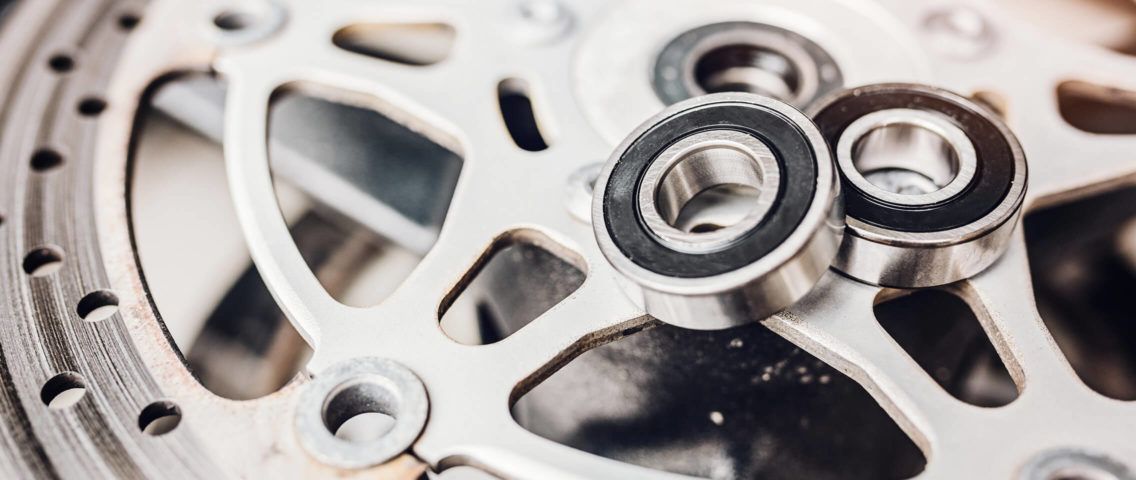
At the best of times managing a spare parts supply chain is a challenge, at worst it can feel like an impossible task. It’s rarely a simple operation. Spare parts are generally stocked at all stages of the supply chain and in multiple locations by manufacturers, wholesalers, retailers, and end users. The number of items is large. Typically, there are thousands, even tens of thousands of different Stock Keeping Units to manage, and units vary in value, lifecycle, weight, size, and criticality. Predicting demand when demand can be so sporadic is seemingly impossible. Add to that equation the high level of service expected and the never-ending battle to bring down costs and the spares supply chain manager’s job begins to feel nigh-on impossible.
Don’t despair! In many cases, it’s possible to increase the efficiency of spare parts management quite dramatically. Automating the parts of the process best done by a specially designed system frees up managers to concentrate on customers, colleagues and tackling the unpredictable, the unexpected and the one-off things that really need a good manager to get them right.
However nearly all the companies that do manage both to improve their quality of service and cut costs have recognised the fact that management systems designed for traditional supply chains generally don’t translate well to the management of spare parts supply. Instead they’ve opted for systems that take into full account the special characteristics of the aftermarket supply chain.
Two Very Different Supply Chains: Aftermarket Vs. Manufacturing
There are key differences between manufacturing supply chains and aftermarket supply chains. Cohen et al. (2006) have summarised them (Table 1).
Manufacturing supply chain | After-sales services supply chain | |
Nature of demand | Predictable, can be forecast | Always unpredictable, sporadic |
Required response | Standardized, can be scheduled | ASAP (same day or next day) |
Number of SKUs | Limited | 15 to 20 times larger |
Product portfolio | Largely homogenous | Always heterogeneous |
Inventory management aim | Maximize velocity of resources | Pre-position resources |
Performance metric | Fill-rate | Product availability (uptime) |
Inventory turns | Six to 50 a year | One to four a year |
In practice, these come down to two main points:
- In spare parts management, it is not possible to reach the same goals as in manufacturing supply chains, nor is it helpful to aim to do so.
- Management concepts developed for manufacturing supply chains typically produce poor results when applied to spare parts supply chains.
Manufacturing supply chains are designed to provide good service levels while maximizing stock turnover. Conversely, spare parts are primarily there to act as insurance when a break-down occurs. In many breakdown situations time lost equals expense. Spares supply chain management is therefore all about stocking items which the end user hopes will never be needed but that when they are needed are required fast. Therefore, maximising inventory turnover is never the main goal in spare parts supply management. In fact, the average turnover in supply chains of companies with a strong reputation for spare parts management is as low as 0.87 (Cohen et. al., 1997).
Improving Spare Parts Logistics
It’ll be no surprise, given the differences between conventional supply chains and those tailored to spare parts supply, that improving spare parts logistics is in large measure about taking the special characteristics of the products and their demand patterns into account. You’ll see these differences most clearly when it comes to demand forecasting, product classification, inventory management, and stocking decisions.
Demand Forecasting
Demand for spare parts is typically sporadic. Patterns where there are individual item peaks alternating with long periods of very low or zero demand present particular challenges for demand forecasting. As a result many widely used forecasting models (e.g. those using moving averages or exponential smoothing) largely based on historical demand averages, do not produce acceptable results. However there are certain forecasting models that are designed specifically to deal with sporadic or intermittent demand that work well. Croston’s model (Croston, 1972), for example, aims to predict both the scale of the demand peaks and the time between them. In addition we can further improve demand forecasting by collecting data on the life-cycles of spare parts and the frequency with which specific faults occur in devices (Ghodrati and Kumar, 2005). However due to the quantity of data needed to make these calculations, this approach is best suited to managing specified components (identified by supply chain managers because of their high cost, sourcing difficulties or other factors) at the application level or for a forecast of a given spare part’s total demand over a particular period.
Even so the accuracy of forecasting of spare part demand is generally lower than for most other areas of retail. It’s essential to recognise this so that investments in improving forecasting reflect the likely returns. It’s more important to plan safety stock levels knowing the limitations that any forecasting model faces.
Classification of Products
The large number of SKUs and multiple stock locations make the inventory management of spare parts complex. Regular manual checking of all the combinations of items and stock locations would consume vast resources.
A widely used approach to simplify product management is the ‘ABC classification’ of products. The ABC analysis is based on classifying products in accordance with their revenue volumes. The idea is to concentrate management resources on the products that offer the biggest margins and the largest profits. However, applying ABC classification to spare parts is often problematic. When product value and other product characteristics vary a great deal, the ABC classification may be misleading. In practice, items as disparate as a short block engine, sold a few times a year, and a butterfly nut, which sells in its tens of thousands, may end up in the same ABC class, although it clearly makes no sense to manage them with the same system and inventory targets.
Making stock decisions according to the ABC classification is also problematic. The top categories often include expensive items with low and sporadic sales and with a very high obsolescence risk. They should not be kept in stock if other distribution options are available. Classification and management based on sales volumes or delivery considerations – ‘XYZ classification’ – is often more effective both from an inventory management and from the end customer’s point of view. Rather than focusing on the average service level, the goal should be to maximize customer satisfaction across all sales.
As in many other product areas, better decisions can be made in spare parts management when the ABC and XYZ classifications are combined. Table 2 illustrates their simultaneous use with an example.
A | B | C | |
X | Aim at as accurate management as possible. Mainly keep items in stock. | Aim at accurate management. Mainly keep items in stock. | Mainly keep items in stock. Purchase items in large batches to decrease handling cost. |
Y | Aim at as accurate management as possible. Keep critical items in stock; make cost-based stocking decisions for the other items. | Keep critical items in stock; make cost-based stocking decisions for the other items. | Mainly keep items in stock. Purchase items in large batches to decrease handling cost. |
Z | Mainly employ a deliver-to-order approach; only keep critical items in stock. | Keep critical items in stock; make cost-based stocking decisions for the other items. | Keep critical items in stock; make cost-based stocking decisions for the other items. |
If there are wide differences in the sales value or volume between different spare parts, it’s doubly important to set appropriate class boundaries. For example, in certain classes it will be important to check that items meet minimum sales quantities, in others that they qualify by total sales value and so forth. The classification boundaries suitable for standard retail goods are seldom directly applicable to spare parts.
Inventory Management
Classifying products properly makes it easier to set effective safety stock levels. Nevertheless, as the right safety stock level for each product depends on factors such as demand predictability, delivery-lead time etc. attaining the ideal inventory level for each item is never possible where products are managed in groups. However the large number of SKUs makes the manual management of inventory control parameters for each item and stock location impractical. The answer lies in automating parts of the inventory management process. Unfortunately, the most popular approaches to calculating item-specific safety stock levels aren’t very well suited to spare parts. One common approach is to set levels of stock coverage to meet expected demand over an agreed period, say three weeks or two months.
However, given that demand for many spares alternates between peaks and extended troughs, using average consumption to set safety stock levels doesn’t work well. In practice, a single peak in demand may exceed the total average consumption over a very long quiet period. The size and frequency of peaks also varies by item, so the safety stock needed for one item might reflect two month’s average consumption, whereas that for another might cover demand for two years.
Commonly used formulas for calculating safety stock levels based on demand variability or forecast error (e.g. Vollman et.al., 1997) are also of limited use when applied to spare parts.
These calculations are typically based on the assumption of normally distributed demand. Yet, demand for spare parts doesn’t usually follow a simple pattern. Demand for some items, especially those that could be considered consumables such as light bulbs, may follow a recognisable and therefore predictable pattern, but managing such items is rarely a problem.
At RELEX we identify re-order points and safety stock levels for spares using simulations. Basically we create a simulation model replicating the inventory replenishment process that takes factors like delivery lead-times and batch size limitations into account. Your real-world demand data, with its natural peaks and slow periods, is used as input data and the software runs multiple simulations to find the inventory control parameters that meet the desired service level targets most effectively. So the nature of the replenishment process, the individual demand pattern of each item and actual lead-times can all be taken into account when optimizing the parameters. The approach has delivered very good results. Take an example: Maatalouskesko – a Finnish company sourcing and selling agricultural production machinery and equipment – has used more accurate inventory management to improve its level of service very significantly without increasing stock levels. Greater automation has also allowed it to reduce its replenishment team and use those human resources elsewhere saving several man-years and considerable expense. (TIEKE, 2007)
Stocking Decisions
Where spares are critical or where long lead times would cause problems it’s necessary to keep stock close to the customer. However, with most items, it’s possible to choose the most cost efficient of several delivery options. That generally depends on demand, value, delivery costs or stocking options. The general rule is that it makes sense to stock cheap items with high sales volumes, but order expensive, rarely sold items only when there is demand. However, product and supplier specific costs often vary significantly and can determine the most efficient delivery option for each specific item. Costs and demand may also change over time, making it necessary to review stocking decisions regularly.
Typically, the choice of delivery channel has a significant impact on logistics costs. It’s essential to factor-in how critical a spare is to a likely customer as well as the cost of the different delivery options. In principle this is simple. The real challenge is to apply it to tens of thousands of products in several locations. In practice, the greatest challenge is producing and keeping the required cost information up to date. Obsolescence is, for example, often a significant risk and cost factor in spare parts, but few companies have exact information on the predicted lifecycles of different products. Information on freight costs at the order line or shipment level is often lacking. The more accurate the cost information available, the better the choices and the greater the savings that can be made. Even rough cost estimates can be used in calculation and still greatly improve decision making.
Time spent collecting and managing cost data pays off. At RELEX we’ve used cost-based delivery channel analysis to produce real benefits for our customers. For example we carried out an item level supply chain analysis for Maatalouskesko that determined which products should be stocked and which should be delivered to order. In practice, large sums can be saved in express freight and other shipping costs when the products are correctly identified for stocking or special order. (GS1 Info, 2007; TIEKE, 2007.)
Conclusion
The number and variety of SKUs make spare parts logistics challenging. As the items are typically so different, efficient management requires that each item and location is individually inspected. Mere generalizations, such as ABC classification, rarely lead to good results. Item-level management enables much better service levels andmore efficient inventories, but requires investment in the quality of cost and demand information as well as system support for product-specific analyses and management.
What Next?
We at RELEX have helped our clients to increase the efficiency of their spare parts management. Using our tools, our customers have been able to improve their service levels and inventory turnover as well as increase the efficiency of their stock replenishment process.
You are interested in making your company more profitable and so are we – contact us already today. An hour’s meeting is enough to go through your company’s situation and to define the first steps towards improving your spares management.
Sources:
Cohen, M.A., Zheng, Y.-S., Agraval, V. (1997), ”Service parts logistics: a benchmark analysis”, IIE Transactions, Vol. 29, pp. 627-639.
Cohen, M.A., Agrawal, N., Agrawal V. (2006), “Winning in the Aftermarket”, Harvard Business Review, May 2006.
Croston J.D. (1972), Operational Research Quarterly, Vol. 23, No. 3, pp. 289-303. Ghodrati, B., Kumar, U., (2005), “Operating environment-based spare parts forecasting and logistics: a case study”, International Journal of Logistics: Research and Application, Vol. 8, No. 2, pp. 95-105.
GS1 Info (2007), ”Automaattinen myymälätäydennys”, 2/2007, pages 6-8. TIEKE (2007), “Kysynnän ennustaminen tuo kaupalle huomattavia säästöjä”,
Vollman, T.E., Berry, W.L., Whybark, D.C. (1997), “Manufacturing planning and control”, 4. edition, pp. 708-709.”