Q&A: Straight answers to AI and data security questions
Feb 21, 2025 • 6 min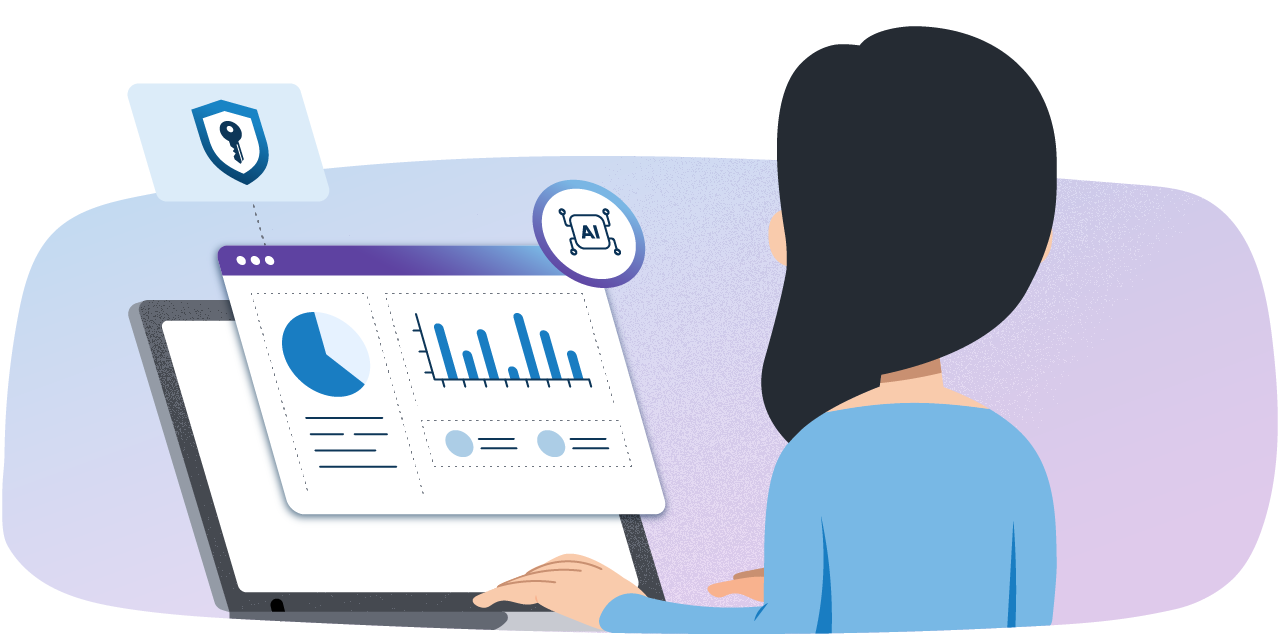
We caught up with Tommi Vilkamo, Director of RELEX AI, to ask the industry’s top-of-mind questions about AI and data security. Here, he clarifies and alleviates the big, vague fears about AI’s data vulnerabilities so companies can determine clear, safe avenues toward success in an AI-driven future.
Q: To start off, Tommi, why are some retail and supply chain companies hesitant to integrate AI into their tech stacks and processes?
Tommi: All this talk of AI generates both excitement and trepidation. AI holds tremendous potential, but business leaders are also cautious about the risks.
Data leaks are the primary concern. Companies are afraid that LLMs and other AI systems will take their proprietary data, train on it, and leak or otherwise expose it to their competitors or the public. Or they’re worried that their trusted supply chain and retail partners might be using untrustworthy third parties, leaving them open to liabilities.
There’s also concern that AI “hallucinations” and miscalculations could result in catastrophic financial consequences.
Other companies I’ve talked to have also mentioned new regulatory requirements and the liability risks there as well.
It’s understandable that companies want to be careful when implementing new (or what seems like new) technology. But a lot of this fear stems from fundamental misunderstandings about what AI is and how it works. If you understand the structure of these AI systems and the models they use, you can better understand where there are seams that could leak data and how to make sure those seams are sealed. You can also see where there is no way for data to escape in the first place and you have nothing to worry about.
Q: So, let’s start with that first knowledge gap. What kind of AI models are we talking about, and what kind of data protection risks might they have?
Tommi: Let’s break it down into three categories. When you’re discussing machine learning, gen AI, LLMs – all of these terms – it helps to know which category you’re talking about.
The three categories are:
- Untrained models
- Trained models
- Pre-trained models
Untrained models are the blank slates. These models hold codes and algorithms but don’t contain any actual data yet. Think of them like a generic blueprint for a house. You have the guidelines to build the house, but you don’t have a house yet. These untrained models are the foundations of, say, ML-based forecasting. The code is there, but it has no information about your company, your sales, or your customers.
Trained models are what you get when those untrained models begin learning from whatever information you feed them and calculating the statistical relationship between different data points. Training the models is like working with an architect or interior designer. As you feed your model input – historical sales data, weather information, expected promotional uplift – you’re customizing your blueprint to your needs and beginning to build the house. And this is your house. Only you have the key. Someone on the other side of town may have used a similar blueprint to build his own house, but he doesn’t have access to yours.
To put it back in the context of supply chain and retail planning, a trained model is, for example, a RELEX customer’s specific instance of the RELEX machine learning models for forecasting. Our customers do not have access to each other’s models because their models are completely separate from each other. Data has no way out of one model and into another.
Pre-trained models operate differently. Large language models by companies like OpenAI, Google, and Meta have been pretrained on data collected from the Internet and other sources. However, the pretrained models are not directly learning anything from the user input. Think of this more like a public library. You visit, get the information you need, then pack up all your data and go. When you leave, the library is the same as it was before you got there. Your visit hasn’t impacted the number, selection, or content of its books.
Of course, the exceptions are untrustworthy providers. But in this regard, AI services are not different from any other cloud service, such as email or storage.
Q: With these categories in mind, then, let’s go back to those concerns you mentioned. Where are the risks of data leaks?
Tommi: The biggest worry among our customers is data usage for training shared models. As I mentioned, RELEX ML models are built specifically for each customer, so there cannot be such data leaks. A customer’s model uses only that customer’s data.
Now, when it comes to data leaks with LLMs, the biggest worry is untrustworthy providers. For enterprise-grade solutions, it’s critical to use only trusted LLM providers, with built-in guardrails, security, privacy, and regulatory compliance.
I’ll use the RELEX AI assistant, Rebot, as an example.
Rebot is built on the Microsoft Azure OpenAI service. While it is powered by OpenAI models, it’s not built on OpenAI services. OpenAI and Microsoft’s Azure OpenAI Service are two different instances providing the same models.
Microsoft never uses their business customers’ data for training, and its OpenAI Service employs Azure’s same enterprise-grade security measures used to protect highly sensitive information for hundreds of thousands of businesses. Whether you’re using Azure or the OpenAI service, you’re protected by the same trusted safeguards.
Rebot functions much the same way. It does not “remember” or train on any customer input. We’re moving rapidly toward integrating Rebot throughout the RELEX solution so customers can generate insights from their own proprietary data – but Rebot does not store this data. It will “call” that data, meaning it will reference it as it runs a calculation if requested, but once that’s done, it hangs up that call and forgets the conversation.
Plus, for those customers still in the “wait and see” camp, you can completely opt out of the RELEX gen AI capabilities at any time.
Q: What about AI hallucinations? How can companies protect against bad data insights that could seriously compromise margins and brand reputations?
Tommi: There’s actually a pretty easy way for companies to prevent AI from spinning out some completely false data that wreaks havoc on their supply chains.
Even the most sophisticated models can miscalculate or “hallucinate,” but this usually stems from a very simple reason – forcing the system to generate a response to something it can’t know. It can only know what’s in its training data, what it can search, and whatever information you provide. If you ask a model something beyond its available information, yes, you might get a hallucination.
That’s why the most accurate and effective AI assistants and agents have strong knowledge bases geared toward a specific industry or task. For instance, Rebot pulls from an exhaustive, comprehensive library of RELEX best practices and solution guides, so it can easily answer a user’s RELEX- and supply chain-specific queries. An AI assistant that doesn’t have that knowledge base and isn’t trained on retail and supply chain documentation won’t be able to answer those questions and is far more likely to generate inaccurate responses.
Also, never forget—we humans are definitely error-prone. We make mistakes, yet human organizations still run successfully because there are checks and balances. With agentic systems, we need to have similar guardrails in place.
Q: Let’s move on to that last concern – regulations. What data regulations are we discussing here, and how do they affect supply chain and retail AI initiatives?
Tommi: There are different regulations coming into effect, mostly notably in the EU.
The EU AI Act is primarily focused on protecting human rights and regulates AI systems according to different risk levels. In supply chain and retail planning, we’re talking about goods, not humans. We’re not handling things like biometric identification, social credit scoring systems, or medical devices. This puts RELEX solutions out of the scope of the act’s “Unacceptable” or “High risk” categories.
The only part of RELEX that currently falls under the act’s purview is Rebot. For this “Limited risk” category that includes AI assistants and chatbots, the act requires that users must know they aren’t talking to a real human. Since RELEX is transparent about the fact that Rebot is an AI assistant, it meets the act’s requirements.
So, in terms of AI regulation, there isn’t really too much that should concern supply chain and retail planners who use our AI-powered systems.
Q: Speaking of Rebot, any future developments you can tell us about?
I think Rebot really exemplifies the astonishing speed and trajectory of AI development we’re seeing in the industry. We’re integrating Rebot into different solutions across the RELEX platform. It was the first supply chain AI assistant on the market, and we’re excited to transform it from generative AI into agentic AI, an AI system that can analyze business data and take actions with increasing levels of autonomy and adaptiveness – but under human supervision.
And we’ve built Rebot for longevity. We’ve even designed it to be LLM-agnostic. If, for any reason, its current foundation LLM service became unavailable or uncompetitive, Rebot could be transplanted onto another LLM so our customers’ business processes wouldn’t skip a beat. We’re excited to be building a system that will grow and develop alongside companies for a long time to come.