The manufacturing demand forecasting revolution: From spreadsheets to AI-powered demand planning
Apr 11, 2025 • 10 min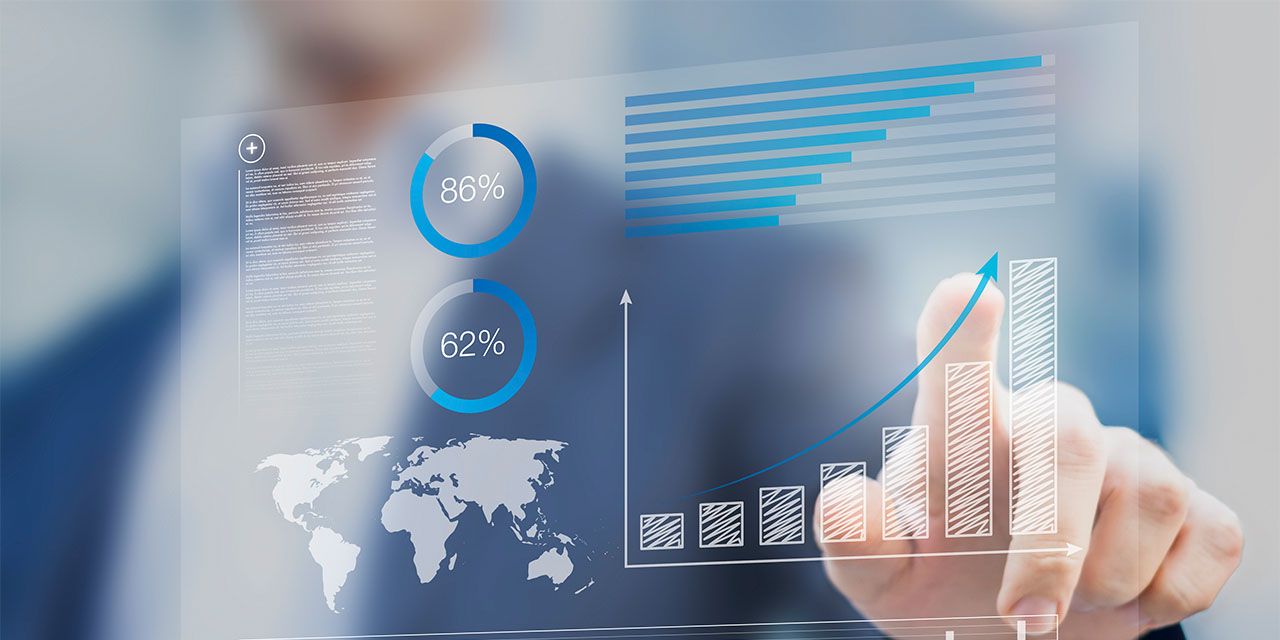
In a landscape of intense competition and shrinking margins, manufacturing demand forecasting and planning have become more strategic than ever. Organizations across both retail and industrial sectors increasingly recognize the critical need for sophisticated tools and processes to navigate market sensitivity and ensure agile decision-making.
And this recognition is well-founded – companies that successfully implement advanced demand planning capabilities achieve significant inventory reductions while maintaining service levels, measurable margin improvements, and enhanced responsiveness to market changes. These tangible business outcomes reflect just how central effective demand planning has become to competitive success.
Over the decades, the role of the demand planner has undergone a remarkable transformation—evolving from basic forecasting into a sophisticated, technology-driven leadership position that shapes core business strategy. What was once considered a supporting operational function has emerged as a central pillar of organizational success.
We’ll examine how this job has evolved, what it has become today, and what we anticipate the demand planner of the future will look like in the coming years.
Note: The timeline references in this article represent approximate periods of enterprise adoption. While some technologies (such as machine learning models) have existed in academic and specialized settings for longer periods, we focus on when they became broadly implemented in enterprise Advanced Planning Systems.
20th Century: The evolution of manufacturing demand forecasting
A brief history: The evolution of demand planning
Before diving into today’s AI-powered landscape, let’s quickly trace the journey of demand planning from its earliest days to the threshold of the current revolution. This evolution reflects not just technological advancement, but a fundamental shift in how businesses view forecasting—from operational necessity to strategic advantage.
The intuition era (Pre-1980s): forecasting as art
In the early days, manufacturing demand planning relied primarily on human judgment and basic calculations. Planners worked with simple moving averages and manual data analysis, operating as “educated guessers” in siloed environments. This approach worked reasonably well in a business climate characterized by simpler product assortments, limited promotional activities, less intense competition, and higher profit margins that could absorb forecasting inaccuracies. During this period, demand planning remained firmly in the background—an operational function with minimal strategic influence.
The statistical era (1980s-2005): The rise of analytical forecasting
The introduction of spreadsheet software and early ERP systems in the 1980s triggered the first major shift in manufacturing demand planning practices. Suddenly, planners had tools to implement statistical methods like ARIMA and Holt-Winters exponential smoothing, transforming intuitive forecasters into data analysts who could select and configure appropriate forecasting models for different product categories.
By the late 1990s, dedicated Advanced Planning Systems (APS) had emerged, offering more sophisticated algorithms and specialized capabilities beyond what ERP systems could provide. Despite computing limitations of the era, these systems represented an important step toward recognizing demand planning as a critical business function. During this period, planning began its migration from back-office operation to business-critical activity as organizations increasingly understood the financial implications of forecast accuracy.
The collaborative era (2005-2020): Breaking down silos
The widespread adoption of Sales and Operations Planning (S&OP) processes marked the next significant evolution, transforming demand planning from an isolated technical function into a collaborative ecosystem. Planners became cross-functional facilitators, orchestrating consensus between sales, supply chain, purchasing, and finance departments. The emergence of Integrated Business Planning further elevated financial considerations in the planning process.
Meanwhile, market complexity intensified dramatically as businesses increasingly relied on promotions and new product introductions to maintain market share. This complexity—coupled with the explosion of SKUs and customization options—strained traditional planning approaches.
For the first time, businesses could forecast at truly granular levels—down to individual SKUs and stores—dramatically improving accuracy and responsiveness. Despite these advances, organizations still struggled with managing overwhelming complexity and breaking down organizational silos while maintaining human planner productivity and effectiveness. This tension set the stage for the AI-driven revolution that would follow.
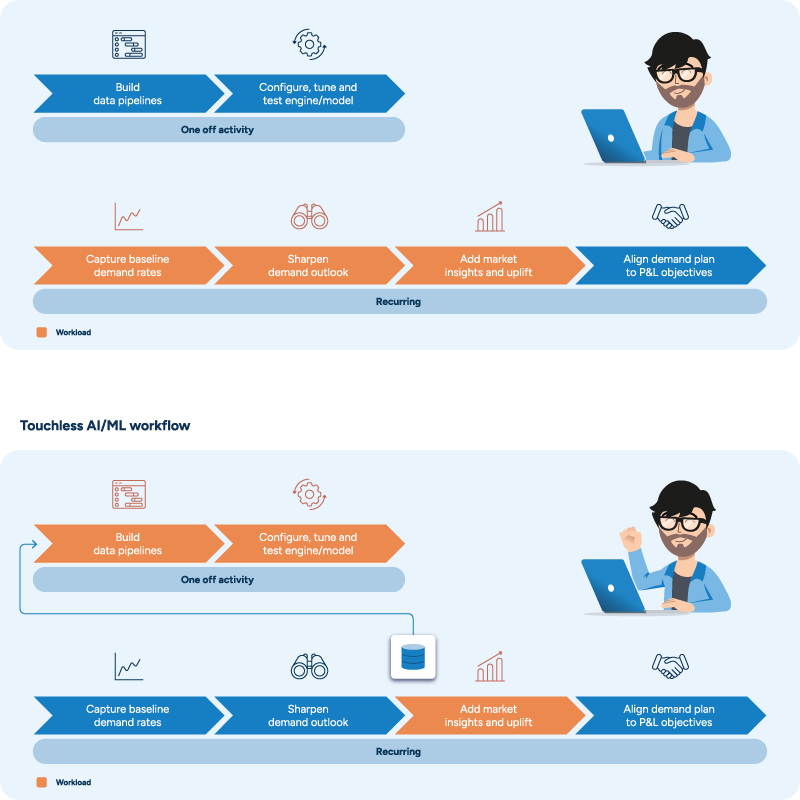
The AI revolution in manufacturing demand forecasting
The introduction of advanced analytics and machine learning technologies, enabled by significantly increased computing capabilities, has fundamentally revolutionized manufacturing demand planning since 2020. Unlike the statistical methods of previous eras, today’s AI-powered forecasting leverages a diverse arsenal of sophisticated techniques:
- Neural networks
- Gradient boosting models
- Reinforcement learning
These technologies have enabled “touchless forecasting”—systems that can automatically generate forecasts based on real-time data and future external factors with minimal human intervention. For example, leading retailers now utilize weather-based forecasting models that can automatically adjust predictions based on upcoming meteorological conditions, translating temperature and precipitation forecasts into expected demand changes at the store level.
Read more: Demand sensing: How to conquer CPG supply chain chaos
The Transformed Role of the Demand Planner
In this new landscape, demand planners are evolving from forecasting specialists into data scientists and business insights experts. Rather than manually reviewing every forecast, they now:
- Explore and integrate external data sources to enhance model performance and provide contextual insights
- Translate technical forecasting insights into business recommendations
- Serve as organizational evangelists for data-driven decision making
- Gain increased strategic influence as their role becomes more central to business planning
- Function as collaborative team players by modeling multiple scenarios and managing complex trade-offs across departments
- Monitor and resolve complex exceptions that AI systems flag as requiring human judgment
This evolution has elevated the demand planner’s position within organizations, transforming what was once seen as a technical support function into a strategic manufacturing demand forecasting advisory role.
The Challenge of Control
Despite the compelling benefits of AI-driven forecasting, many organizations face a significant psychological hurdle: the fear of losing control. This manifests in several ways:
- Trust issues: “Black box” algorithms that produce accurate results without clear explanations of their reasoning can create skepticism among stakeholders
- Skills transition anxiety: Planners who have built careers on statistical expertise may feel threatened by systems that automate previously valued skills
- Accountability concerns: Questions about who is responsible when AI-generated forecasts lead to business decisions with negative outcomes
Successful organizations address these concerns through:
- Implementing explainable AI systems that provide transparency into key drivers behind forecasts
- Creating structured transition programs that help planners develop new skills in AI oversight and business analysis
- Fostering domain expertise development and building closer customer relationships to provide more effective guidance
The Power of Exception-Based Management
Establishing clear governance frameworks that define roles and responsibilities in AI-augmented planning processes has become essential as demand planning grows more complex. This implementation of control strategies enables effective exception-based management—now the focal point of modern demand planning.
With expanding data volumes, planners rely on AI systems to identify and prioritize exceptions based on business impact, allowing them to focus on issues that significantly affect inventory, service metrics, and financial performance.
AI-powered planning systems have evolved to not only process vast amounts of data but also to explain the reasoning behind key insights, enabling deeper root-cause analysis and more strategic decision-making. By filtering out noise and highlighting critical deviations, AI helps planners focus on the most impactful issues, enhancing both efficiency and accuracy in forecasting.
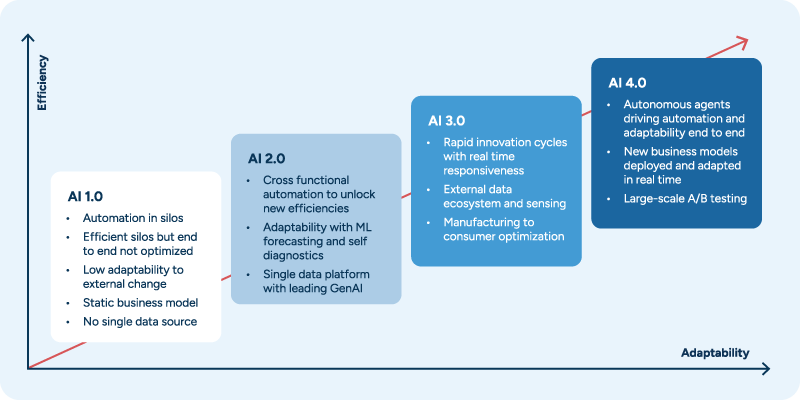
Future Outlook: AI assistants and system-to-system collaboration
The remarkable advancements we’ve witnessed in demand planning thus far represent only the beginning of a much larger transformation. Just as Cloud computing and SaaS solutions redefined planning in the previous technology wave, the emergence of Agentic AI marks the next evolutionary leap for demand planners—a shift that promises to be even more profound.
Today’s AI assistants, exemplified by tools like ChatGPT and Gemini Professional, provide merely a glimpse of what’s to come. These systems are evolving at an unprecedented pace, with AI assistants transforming into increasingly autonomous agents with sophisticated decision-making capabilities that will fundamentally reshape the world, including demand planning as we know it.
For forward-thinking demand planners in manufacturing demand forecasting, this evolution will transform the profession into a more rewarding and intellectually engaging discipline, driving improvements across three critical dimensions:
Unprecedented efficiency
The future of demand planning will move beyond today’s exception alerts with manual resolution to a model of exception management with automated resolution. We anticipate a dramatic reduction in repetitive manual tasks as AI agents develop the capability to independently perform exception-resolution or exception-solving tasks.
Consider this practical example: If a demand planner consistently corrects promotional uplift in a specific way— solving 9 times out of 10 the exception the same way. Future AI systems will recognize these patterns and proactively apply the appropriate corrections.
Rather than manually implementing the same solution repeatedly, planners will simply validate the AI’s auto-correction, dramatically improving throughput and allowing focus on more strategic activities. Scenario planning will become the “bread and butter” of demand planners—the standard feature most frequently utilized to evaluate different outcomes. Additionally, a meta-exception process will emerge, where planners periodically review patterns in AI-corrected exceptions to ensure the system continues to make appropriate adjustments and to identify opportunities for further refinement.
Enhanced auditability and transparency
Future AI agents will transcend basic data processing to become true analytical partners. They will not only identify key parameters requiring attention but will also contextualize insights in ways that enhance human decision-making. These systems will provide deeper explanations of their reasoning, helping validate corrections and supporting more informed choices.
The evolution of Collaborative Planning, Forecasting, and Replenishment (CPFR) will undergo a parallel transformation. Traditional CPFR focused on retailer-supplier data exchanges requiring significant human mediation. The future will be characterized by true System-to-System forecasting—AI-powered platforms autonomously exchanging data and refining forecasts with minimal human intervention. This will lead to superior demand signal quality.
The quality of demand forecasting will continue to improve through two parallel developments:
- Computational and algorithmic advancements: Enhanced computing power coupled with breakthrough research will drive continuous improvements in model quality and capabilities.
- Revolutionary data acquisition and preparation: Even the most sophisticated models are constrained by the quality of their inputs—”garbage in, garbage out” remains an eternal truth. Next-generation AI tools will revolutionize data gathering and preparation, providing a more accurate and holistic understanding of market conditions and potential demand drivers.
These advancements will deliver particularly powerful capabilities for assortment optimization:
- Enhanced inter-product analysis: AI systems will develop sophisticated understanding of product interactions through optimized basket analysis, revealing previously invisible cannibalization and halo effects.
- True customer-centric and best-in-class new product forecasting: In an increasingly connected world, demand planning will leverage unprecedented market intelligence. Systems will analyze pre-sales activities and consumer behavior across digital ecosystems to anticipate demand shifts before they materialize in traditional sales data.
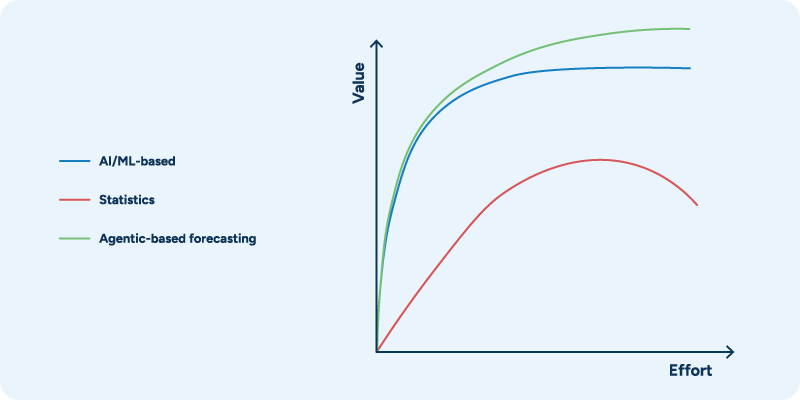
Organizational implications
As organizations continue their transition to data-oriented, analytical mindsets, the demand planner’s role will become increasingly central. However, this evolution won’t occur in isolation—other functions are simultaneously transforming as well.
The most agile organizations may evolve toward integrated roles that merge demand and supply planning functions, creating a unified position focused on end-to-end optimization and customer-centric trade-offs. The demand planner of the future will be an “agentic ambidextrous planner”—a professional who collaborates seamlessly with AI systems while maintaining an end-to-end perspective on the business, acting more as a strategic troubleshooter than a tactical forecaster.
Beyond simply correcting forecasts, future demand planners will develop expertise in assessing and optimizing the entire forecasting process within their organizations. This higher-level understanding will involve evaluating how forecasting should be structured, which methodologies are most appropriate for different product categories, and how to continuously improve organizational forecasting capabilities.
When we consider demand planning in the broader context of an AI-powered world, there are even more possibilities. AI-based knowledge transfer applications can rapidly upskill professionals with industry-specific expertise—whether in dairy, cable manufacturing, pharmaceuticals, or other specialized sectors. These tools can compress years of domain knowledge acquisition into weeks, allowing planners to quickly develop deep understanding of the unique demand patterns and constraints within specific industries.
In the supply chain planning software landscape, we may witness a “winners take all” concentration of capabilities. In this competitive environment, success will likely come to vendors who deliver:
- Highly agile implementation processes paired with problem-solving microservices that address specific business challenges
- Strong customer advocacy built on proven ROI and measurable business outcomes that create lasting platform loyalty.
- Lasting relationship with software partners leveraging customer-centric teams able to transfer learnings and best practices from AI
Learn more: CPG supply chain trends
The future belongs to the strategic demand planner
The evolution of manufacturing demand planning reflects a remarkable journey—from intuition-based estimations to sophisticated, AI-powered strategic processes. This transformation mirrors broader technological and organizational trends while highlighting the increasing strategic importance of accurate demand forecasting in driving business success.
As we’ve seen, the demand planner’s role has continuously adapted to new challenges and opportunities:
- From isolated guesswork to collaborative consensus-building
- From simple statistical methods to advanced machine learning algorithms
- From tactical forecasting to strategic business guidance
Today’s demand planners stand at another inflection point. And while significant, this inflection point is unlikely to be the last one. Future technological and organizational shifts will likely create additional inflection points, occurring with increasing frequency as the pace of change accelerates.
As organizations continue to embrace automation and advanced analytics, the role will increasingly focus on strategic oversight rather than operational execution. The future state envisions a landscape where human planners collaborate with AI systems to enhance decision-making while navigating the complexities of modern supply chains.
For businesses, the implications are profound. Companies that successfully integrate these emerging capabilities into their planning processes will gain significant competitive advantages through:
- More accurate forecasts leading to improved inventory management
- Enhanced responsiveness to rapidly changing market conditions
- Better alignment between demand signals and broader business strategy
- More efficient resource allocation across the organization
For individual planners, this evolution presents both challenges and opportunities. While certain technical skills may become less relevant, the demand for professionals who can bridge the gap between AI systems and business strategy will only increase. Tomorrow’s demand planning leaders will combine technological fluency with deep business acumen—a powerful combination that will elevate the profession to new heights.
Embracing this transformation will be essential for organizations aiming to remain competitive in an increasingly complex and fast-moving market landscape. The demand planners who successfully navigate this evolution will find themselves not just forecasting the future—but actively shaping it.