Use AI to make messy datasets useful, not perfect
Nov 1, 2024 • 5 min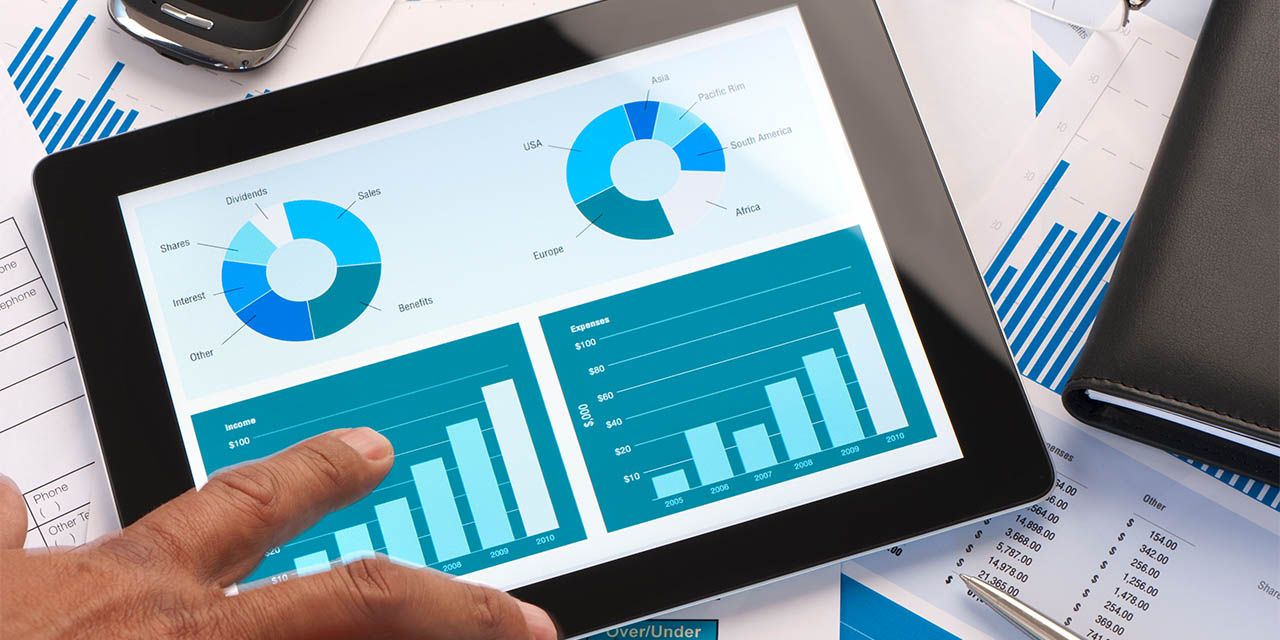
One RELEX survey showed that 56% of respondents ranked data quality as the biggest challenge in implementing AI in retail and supply chain management.
This is no surprise. Data quality is one of the key pillars of data governance, and most people looking into AI implementations are aware of its importance. “AI is only as good as the data it’s trained on,” as the saying goes. But this maxim isn’t complete or nuanced.
The pervading assumptions about AI and data raise two issues. First, most people see the connection between the two as unidirectional, with data feeding AI. Really, this link should be a two-way street, with each component supporting and refining the other. In other words, you can deploy AI to improve its inputs and outputs.
The second issue is analysis paralysis. Planning leaders must deal with real-world datasets. These datasets are messy and chaotic and sometimes exhibit unexplainable behaviors. This problem can seem insurmountable. The temptation is either to assume nothing can be done and accept sub-optimal processes or to be hamstrung by unrealistic data goals, thinking that data must be perfect to be usable and aiming for quality that can only exist as a theoretical ideal.
AI is only as good as the data it’s trained on, yes. But getting data you can use is more important than getting perfect data. Your data record will never be flawless, but the right AI approaches help you refine, trust, read, and act on that data. A pragmatic approach uses AI to accommodate data gaps, errors, and inconsistencies and achieve high – and operative – data quality standards, maximizing accuracy and delivering timely, real-world benefits.
Data quality can be considered from two angles. One angle is concerned with technical correctness, handling problems like data entry errors or missing history. The second focuses on business value, making sure that data and processes are aligned and generating outcomes that make sense.
If data quality is the foundation of successful AI-powered supply chains, these tools ensure that foundation is solid.
Data cleansing
Data cleansing lies in the “technical correctness” category.
Supply chain data can be riddled with mistakes. Minute errors like typos or incorrect values, duplicate records, and missing data points can send negative ripple effects throughout the system.
Data cleansing techniques can spot and correct those errors, remove duplicates, and fill in those gaps using statistical methods or domain knowledge. They also standardize data formats to make it easy to compile, share, and analyze data across the organization.
Advanced machine learning can compensate for anomalies and alert planners to data inconsistencies that would otherwise go unnoticed. For instance, advanced systems can detect missing promotional data and highlight exceptions by detecting unexplained sales uplifts that have the characteristic profile of a campaign. This is particularly important during the pre-processing phase of an implementation. Users can then ensure the correct master data is in place and therefore further boost the accuracy of forecasts.
In some cases, AI-powered predictive models can be used to create a synthetic view of data that is more accurate and reliable than actual inventory records.
Outlier detection
Outlier detection brings us out of the technical focus of data cleansing into the realm of business-related AI strategies.
Sometimes, data points land far outside the scope of the rest of the dataset. This could be traced to a simple error, or a promotion at the store level causing a sales spike, or a barge getting stuck in the Suez Canal and causing massive delays. Other times, these data points will never be explained or repeated. Perhaps someone was hosting a massive 50th anniversary party and bought every steak in the store. Planners could not have seen this coming, but it’s an outlier, not a data error, even though it’s not representative of normal demand conditions. These outliers can wreak havoc on analyses and forecasts. Outlier detection stops these stray data points from skewing forecast calculations for a more reliable picture of demand.
Noise detection
Not all data points are created equal. Even if every record is accurate and correct, some data is just noise – random fluctuations or irrelevant variations that muddy true demand signals and complicate demand planning.
Every data point is influenced by some random variation, minor or major, that cannot be tracked back to its root cause and therefore, cannot be “learned” by an algorithm. For instance, looking at all the data points in a set time period for one product in one store is very noisy. The effects of all the atypical influences are fully visible, but there is no way to differentiate them from significant demand signals. However, looking at the average behavior for the same product across 20 stores reduces this noise level significantly; the random variations cancel out, and the true pattern emerges.
Pooled models, which combine the data of similar products with little or no data of their own, give the algorithm more points of reference. The increase in reference depth allows it to distill this information and identify what is typical and significant to the overall demand pattern for these product types.
Level shift detection
Level shift detection uses machine learning (ML) to identify and cope with major (and often unexplained) step changes in demand – something forecasting models have struggled to do historically. ML algorithms analyze demand patterns over time at the location-specific level. They then calculate average daily sales before and after potential shift dates to see if shifts have occurred. If so, they incorporate them into the forecast to improve accuracy, which is especially helpful for slow-moving items with intermittent or lumpy demand.
Let’s take wholesale as an example. Wholesalers typically have a smaller number of customers than retailers, but those customers are ordering large volumes. Wholesale customers often exhibit stop-and-go ordering, pausing orders without warning and just as suddenly resuming them, depending on changes to their own business requirements and processes. These unpredictable ordering shifts lead to chunky step changes in demand.
For a retail example, let’s say a highly anticipated book sees significant sales upon launch. Future sales, however, will be dictated by the book’s continued popularity, which may be influenced not only by the writing quality but also by reviews or sudden social media trends. On the other hand, a book may see very little traffic upon release, but social commentary or a single viral review can bring about skyrocketing sales. In this case, planners would only see these correlations after the fact, since virality would be impossible to predict. Level shift detection helps them track these shifts and plan accordingly.
In other cases, planners may remain completely unaware of the cause of a sudden shift. Perhaps there was construction in the parking lot of a store, making it more difficult to access. Planners don’t have that level of visibility but can see a two-week sales drop in the history of that store. Even if they can’t correlate that shift to an event like a promotion, price change, or weather, those shifts still exist and need to be accounted for to maintain a robust forecast.
Trustworthy data delivers ROI and underpins a successful AI strategy
Trust in data translates to trust in the planning system. The solution generates higher-quality output with fewer manual corrections and less planner oversight, creating the sort of domino effect business leaders like to see. Better data means improved planning and inventory efficiency, wider margins, and higher service levels and customer satisfaction.
And after all, AI isn’t just about fixing data issues; once you can achieve and maintain data of that quality and accuracy, it becomes a dynamic part of your overall AI strategy. The better your data, the more you can do with it and the more resilient you can make your supply chain.
Just as the techniques above are the best suited to cleaning up data, there is a plethora of AI capabilities specifically developed for retail and supply chain industries. It’s about applying the right AI approach to these datasets to meet each industry challenge. With diverse AI portfolios, companies can implement innovations rapidly to remain competitive and profitable far into the future.
Data is just the first step.