Why a synthetic inventory is probably better than your real inventory
Oct 21, 2024 • 5 min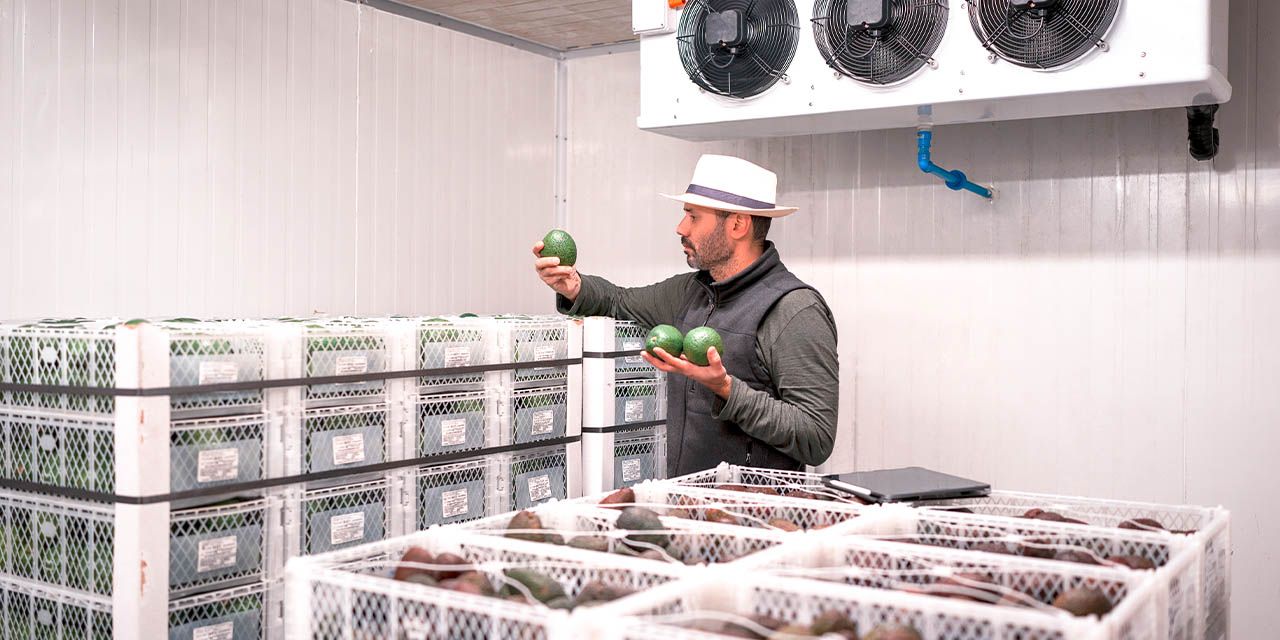
There are two universal truths about inventory data. One, accurate inventory data is the backbone of maintaining smooth operations and proper demand forecasting. Two, most inventory data is probably not all that accurate.
Due to a wide variety of inventory errors (human or otherwise), most stores struggle to maintain a true understanding of their inventory. This lack of visibility leads to a significant issue: the data that retailers rely on for demand forecasting is often inaccurate or incomplete.
And that’s the core of the problem. When the data entering your forecasting system is garbage, the plans that come out of it will be garbage, too.
The irony is that poor data accuracy is widely recognized among retailers as the number one issue leading to stock-outs. That means that data corruption isn’t just a minor inconvenience; it is a widespread and well-known problem that affects the entire retail industry.
So how can you order to your store when you don’t truly know what is in it? Well, the first step is accepting your data is a problem, one preventing you from getting better. And the second? Adopting a synthetic stream of data that is better than the one in your system.
The problem with current inventory data
Before we delve into creating synthetic inventory and its advantages over typical store data, we must understand why data is error prone. How did data end up so full of errors that we’d recommend just throwing it out?
One of the most pervasive issues resulting in inaccurate inventory data is the phenomenon known as phantom inventory. And the issue is just as spooky as the name suggests.
Imagine the panic when the system registers 50 units of hamburger meat, but the store runs out at 20—right before the most popular cookout weekend of the year.
This discrepancy can arise from several causes, each contributing to the illusion of stock that doesn’t actually exist.
Causes of phantom inventory
- Unrecorded movements: When items move within the network without updating the inventory system, the misalignment causes stock discrepancies. This issue also includes instances where the system records movements that didn’t occur, such as stock not being picked or delivered as indicated.
- Breakage: When damaged goods are not adequately accounted for, they create false records of available inventory.
- Spoilage: Fresh and perishable items might spoil or develop mold, leading to early stockouts. The issue arises when staff inaccurately record spoilage, causing data discrepancies.
- Theft: Shoplifting or internal theft can result in missing items that still appear in the system as available stock.
- Data entry errors: Simple mistakes during data entry, such as incorrect quantities or mislabeling, can lead to significant inaccuracies.
- Fraud: Intentional changes to inventory records create phantom inventory. This problem can occur through mis-weighing or mis-scanning products, which retailers often view similarly to theft.
These issues might seem like little one-off nuisances on their own. But these little errors can accumulate faster than you think, creating a distorted picture of what inventory is actually in your store.
This inventory drift can lead to significant discrepancies between recorded and actual stock levels. The longer those problems go uncorrected, the bigger the gap between your system and actual inventory becomes. Phantom inventory can cost a retailer up to 3% of revenue!
Once again, if you attempt to make your ordering plan based on stock that isn’t there, you’ll be in trouble when the inventory shipment arrives.
So, if the traditional ways aren’t working what can you do instead? To fully address this, retailers must look beyond conventional methods and consider innovative solutions that provide more accurate and reliable data.
Read more: Distinct AI approaches to three top business concerns
Introducing a “true” inventory
Given the challenges posed by phantom inventory, it’s clear that traditional methods of inventory management are no longer sufficient. However, a predictive inventory leverages advanced technologies, such as machine learning and artificial intelligence, to create a synthetic inventory that is more accurate and reliable than actual physical counts.
Synthetic Inventory: A predictive inventory system that generates synthetic inventory data effectively mirrors real-world stock levels with greater accuracy. This approach analyzes patterns in historical data and real-time store-level inputs to predict and correct discrepancies that would otherwise go unnoticed.
This technology enables the demand planning system to learn from past data and identify potential issues before they become significant problems. For instance, it can detect anomalies that suggest phantom inventory, which typically means drops in stock levels that don’t align with sales data.
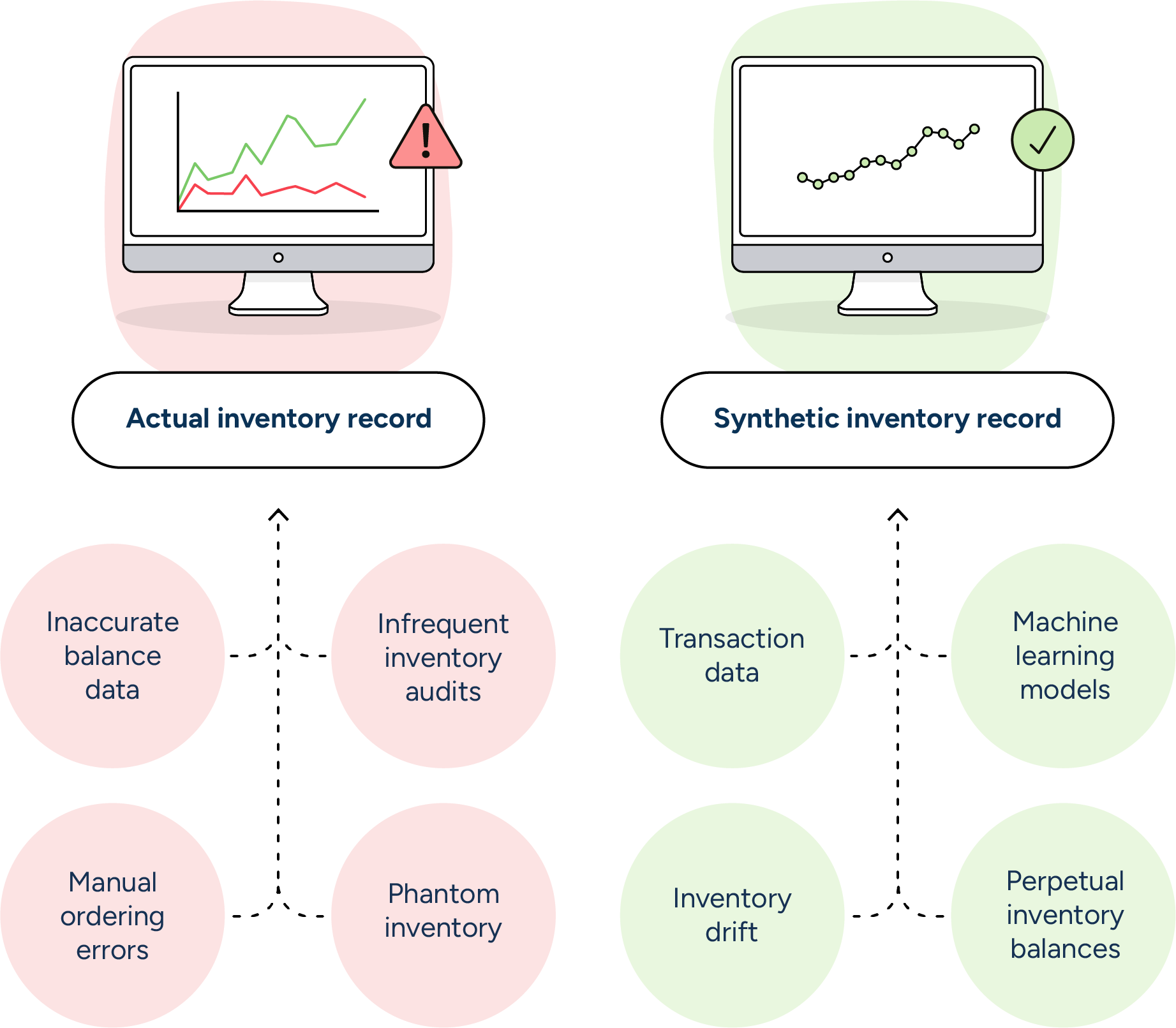
Benefits of a synthetic inventory
- Improved accuracy: By continuously learning and adapting, predictive inventory systems provide a more accurate representation of stock levels, reducing the risk of stockouts and overstocking.
- Proactive issue detection: Machine learning algorithms can identify and flag potential inventory issues, allowing retailers to address them proactively rather than reactively.
- Enhanced efficiency: With more reliable data, retailers can optimize their ordering and stocking processes, leading to better demand planning and reduced store operational costs.
A great example of where a predictive system can shine is in omnichannel retailing. Omnichannel operations survive on confidence in inventory data. Predictive inventory ensures that the data driving these operations is as accurate as possible, enabling seamless integration across various sales channels.
Predict the future instead of fear it
The key to better inventory management is embracing predictive inventory systems. With the help of machine learning and AI, you can create synthetic inventory data that far exceeds the old-school methods. If AI can help you identify and fix inventory issues faster, why wouldn’t you use it?
These synthetic inventory systems not only tackle the pesky problem of phantom inventory but also boost efficiency, cut down on stockouts, and keep your customers happy.
So, next time you’re puzzling out another stockout issue, remember: A synthetic version of your inventory data is probably better than your current one.